Introduction
Samgyetang and Baeksuk are traditional Korean soups that are prepared using whole juvenile chickens filled with garlic, glutinous rice, jujube, and ginseng to combat the detrimental effects of the summer heat (Jayasena et al., 2013). Considering its short and concentrated seasonal demand, Samgye is generally prepared and distributed using meat from white semi-broiler (WSB) chickens, which are crossbred using a male broiler and female laying hen (Jeong et al., 2020). WSB is characterized by their white feathers, smaller size, and reduced fat and account for 60% to 70% of the Samgyetang market (Cho et al., 2007). This commercial dominance is attributed to aspects of WSB like a cheaper price, higher stability during high-temperature processing, and a chewy texture compared to commercial broiler (Park et al., 2011). However, this is an unofficial breed raised by private industries and several safety issues have recently been put forth regarding its production process (Jeong et al., 2020). Korean native chicken (KNC), is a slow-growing breed and is known for possessing lower fat content and a firmer texture than WSB and commercial broiler chickens (Jeon et al., 2010). KNC also has a unique flavor and a chewier texture when compared to commercial breeds and are known to contain higher concentrations of amino acids and inosinic acid, contributing to their umami taste (Jayasena et al., 2013; Jayasena et al., 2015b). The complex flavors of KNC can result from the combination of various inherent compounds including arachidonic acid (C20:4), glutamic acid, inosine 5’-monophosphate (IMP), and endogenous bioactive compounds such as anserine, creatine, and carnosine (Jayasena et al., 2013; Jayasena et al., 2014a; Jayasena et al., 2015b; Jung et al., 2013). These dipeptides are known to possess antioxidative, antiaging, pH-buffering effects, and closely related to energy metabolism (Lee et al., 2015).
While metabolomic analyses are generally used to explain changes in the physicochemical properties of various meat products and rely on various chemometric analyses (Kim et al., 2020a; Kim et al., 2020b; Simmler et al., 2014), there has been an increase in the number of nuclear magnetic resonance (NMR)-based studies used to evaluate the metabolic profile of these meat samples without evaluating the chemical characteristics of the target metabolites (Simmler et al., 2014). NMR-based analysis combined with multivariate analysis was used to understand and/or to elucidate metabolic changes of meat samples (Kim et al., 2020a; Kim et al., 2020b). Several studies have used NMR-based analysis of meat samples to determine their metabolic profiles, and many of these profiles have been used to differentiate meat samples based on breed, age, geographical origin, and metabolic fingerprint as determined by one-dimensional 1H NMR (1D 1H NMR) analysis (Beauclercq et al., 2016; Jung et al., 2010; Kodani et al., 2017; Straadt et al., 2014). However, 1D 1H NMR analysis may not reveal all the differences in the metabolic profiles as there may be chronic overlap in the obtained data (Kim et al., 2020a). To overcome this limitation, many studies have opted to include two-dimensional NMR (2D NMR) to solve the issue of overlapping via dimensional expansion (Kim et al., 2020b). 2D NMR qualifies and easily quantifies the specific peak exhibited by various metabolites which can then be used to evaluate quality-related metabolites and breed authenticity when used in conjunction with machine learning algorithms (Dass et al., 2017). Machine learning algorithms allow researchers to use the complex data obtained from various chemometric analyses to rapidly classify samples into specific groups without generating experimental bias (Jiménez-Carvelo et al., 2019).
The objective of this study was to evaluate and quantify differences in the taste-active and bioactive compounds in different breeds of chicken and to determine if these values could be applied to a machine learning algorithm and used to distinguish between different breeds of chicken, KNCs (-A, -C, -D, and -H) and WSB, based on metabolic differences in their breast and thigh meat.
Materials and Methods
Deuterium oxide (D2O), D2O with 3-(trimethylsilyl)propoinic acid-2,2,3,3-d4 (TSP) sodium salt, and mono- and di-phosphate sodium salts (anhydrous form) were purchased from Sigma-Aldrich (St. Louis, MO, USA). Potassium hydroxide was purchased from Daejung Chemicals & Metals (Siheung, Korea).
KNCs [newly developed KNCs (KNC-A, -C, and -D) and commercial KNC-H] and WSB were raised under the same conditions for 5 wk at a pilot-scale farm (Gimje, Korea). All chickens were raised across 15 pens (25 chickens/pen) within a single house and food and water were provided ad libitum throughout the entire experimental period. After 5 wks, the chickens were transferred to a slaughterhouse (Iksan, Korea) and held in lairage overnight. The slaughter process then proceeded automatically. Chickens were stunned in an electrical water bath, de-feathered, eviscerated, and air-chilled. Eight chicken carcasses (2 chickens/pen) of similar size (800±50 g) were randomly selected and deboned, vacuum-packaged, and transferred to the laboratory (Seoul, Korea) using a cooler filled with ice. Eight samples (comprising both breast and thigh meat) were ground using a meat grinder (MG510, Kenwood Appliances, Dongguan, China) and homogenized. Subsequently, three homogenized samples (weighing approximately 100 g each) were then collected, vacuum-packed, and stored at –70°C for further analysis. All frozen meat samples were thawed at 4°C for 24 h before analysis.
Thawed ground chicken meat (5 g) was homogenized at 1,720×g for 30 s (T25 basic, Ika, KG, Staufen, Germany) in 0.6 M perchloric acid and then centrifuged (Continent 512R, Hanil, Incheon, Korea) at 3,086×g for 15 min. The supernatant was transferred into a new test tube and neutralized using potassium hydroxide. Neutralized extracts were centrifuged again under the same conditions and then the supernatant was filtered (Whatman No. 1, Whatman PLC., Brentford, Middx, UK) and lyophilized (Freezer dryer 18, Labco Kansas City, MO, USA) before storing at –70°C until further use in NMR analysis.
These lyophilized extracts were reconstituted using 1 mL of 1 mM TSP D2O (20 mM phosphate buffer, pH 7.0), placed in a water bath at 35°C for 10 min, and then centrifuged at 3,086×g for 20 min at 4°C. The supernatants were then transferred into a microcentrifuge tube and centrifuged at 17,000×g for 10 min. The supernatant (600 μL) was then transferred into an NMR tube prior to NMR analysis.
1D 1H NMR and 1H-13C heteronuclear single quantum coherence (HSQC) were recorded in D2O at 298 K using the Bruker 850 MHz cryo-NMR spectrometer (Bruker Biospin GmbH, Rheinstetten, Germany). 1D 1H NMR was performed by applying a modified standard zg30 (recycle delay of 1 s) default in Topspin 3.6.2 (Bruker Biospin GmbH). The 1D 1H NMR experiment was performed using 64 k data points, a sweep width of 17,007.803 Hz, and 128 scans. The 1H-13C HSQC experimental conditions were as follows: 2 k data points in the t2 domain and 512 increments in the t1 over eight scans with a spectral width of 11 ppm for the f2 dimension and 180 ppm for the f1 dimension; and a coupling constant value of 145 Hz designed to set delay durations for short-range correlations. After acquisition of data, NMR baseline correction was performed manually and the TSP reference was used to align the spectra obtained from both 1D 1H NMR and 2D 1H-13C NMR.
Metabolite peaks were identified using standard compounds, the human metabolome database (HMDB; hmdb.ca), and the biological magnetic resonance bank (BMRB; bmrb.wisc.edu). Only peaks with no and/or slight overlap were considered for quantification. Metabolites in the 1D 1H NMR analysis were quantified using methods described by Kim et al. (2019). Prior to multivariate comparison, peak intensities in the 1H-13C HSQC data were quantified using AMIX (Analysis of MIXtures software v3.9, Bruker Biospin GmbH) according to the methods described by Kim et al. (2020a).
Statistical analysis for taste-active and bioactive compounds was performed using the procedure of the general linear model for comparison of quantified metabolites obtained from 1D 1H NMR in each meat portion. Significant differences among the mean values were determined by using Duncan’s multiple range test and the SAS software (SAS 9.4, SAS Institute, Cary, NC, USA) with a confidence level of p<0.05. All experimental procedures were conducted in triplicate.
A total of 44 integrated metabolite peaks (arbitrary units) from the 1H-13C HSQC dataset were considered for multivariate analysis. Prior to multivariate analyses, the absolute intensities were log-transformed (generalized logarithm transformation) and auto-scaled (mean-centered and divided by the standard deviation of each variable). Datasets [30 (samples)×44 (metabolites)] were then analyzed using one-way ANOVA and the Tukey’s post hoc test (p<0.05) for comparison of chicken breeds in each meat portion (breast and thigh). Principal component analysis (PCA, R mode), partial least square-discriminant analysis (PLS-DA), and biomarker analysis were performed using Metaboanalyst 4.0 (www.metaboanalyst.ca), and a heatmap analysis of the top 30 metabolites identified using ANOVA was completed using Euclidean distance and Ward’s cluster algorithm. Multivariate receiver operating characteristic (ROC) curve analysis was completed using the linear support vector machine (SVM) classification, and its features were ranked based on the SVM built-in ranking method.
Results and Discussion
Taste-active and bioactive compounds in the breast and thigh meat were quantified using 1D 1H NMR analysis as numerical quantification of 2D NMR spectra requires standard curves for each compound (Fig. 1; Kim et al., 2020b). The composition and quantity of each of these taste-active and bioactive compounds were observed to be breed-specific (Table 1), with the breast meat from KNC-D demonstrating the highest levels of aspartic acid, glutamic acid, carnosine, creatine, and IMP among meat samples obtained from all breeds (p<0.05). KNC-C had the highest anserine content (p<0.05), while KNC-A demonstrated the lowest quantity of any of the examined flavor-active compounds (p<0.05). Aspartic and glutamic acid are both free amino acids and their presence is markedly associated with the meat flavor, which may be attributed to their synergistic effect with IMP that enhances the umami flavor associated with high-quality meat products (Dashdorj et al., 2015; Jayasena et al., 2014b; Yamaguchi, 1967). The amount of free amino acids in chicken meat could be proportional with growth rate of chicken breed (Ali et al., 2019). Likewise with previous study, the amount of glutamic acid and aspartic acid may present proportionally among KNCs since KNC-A showed the lowest growth rate (Lee et al., 2018). Anserine and carnosine both share a histidine base and are commonly referred to as histidine dipeptides. Anserine is predominantly found in poultry and can be synthesized from carnosine via carnosine N-methyltransferase activity (Jung et al., 2013). These histidine dipeptides demonstrate multiple activities including exhibition of bioactive functions and act as taste-active compounds enhancing the underlying umami taste of meat (Dashdorj et al., 2015; Lee et al., 2015). Creatine is one of the major metabolites produced during rigor mortis (Watabe et al., 1991) and plays a vital role in energy metabolism, with its concentration often being inversely proportional to meat quality (Jung et al., 2013). These bioactive compounds could be varied by the characteristics of breeds such as enzyme activities, resulting in different levels of bioactive compounds (Jung et al., 2013). Following death, adenosine 5’-triphosphate is rapidly degraded to adenosine 5’-monophosphate (AMP) resulting in energy depletion, and is then converted to IMP by AMP deaminase, thus increasing the umami flavor of the meat (Dashdorj et al., 2015). In previous studies, nucleotide levels was also proportional to bird’s age (Jayasena et al., 2015b; Xiao et al., 2019). Therefore, KNC, which has relatively lower growth rate showed higher nucleotides levels than those of broilers (Kim et al., 2020b). In the present study, the breast meat of KNC-D and -H had higher IMP levels than WSB and other KNCs. However, that of thigh meat showed the highest in WSB, which need to investigate further. Newly developed KNC-D demonstrated an overall improvement in the concentration and composition of both taste-active and bioactive compounds in the breast meat when compared to commercial KNC-H and WSB breeds. Additionally, the aspartic acid, glutamic acid, anserine, and creatine content in the thigh meat was highest in KNC-C and lowest in KNC-H (p<0.05) (Table 1).
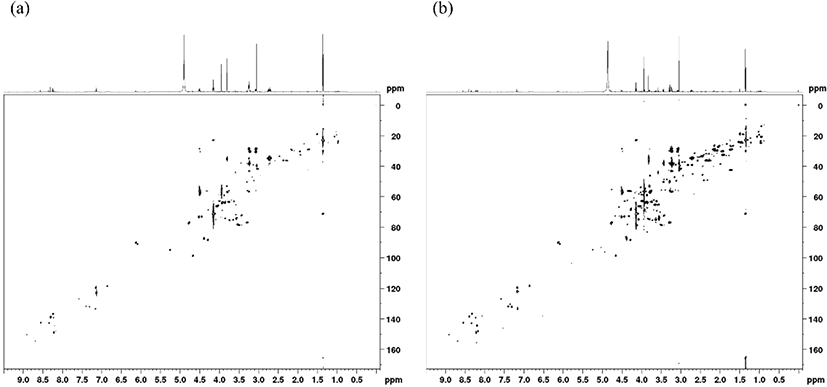
The aspartic acid and glutamic acid levels were lower in the breast meat samples compared to those in the thigh meat samples (p<0.0001; Table 1). However, the anserine, carnosine, creatine, and IMP contents were higher in the breast meat (p<0.0001). These results are consistent with those reported in previous studies that show that breast meat has higher carnosine content than thigh meat (Jung et al., 2013). The amount of carnosine can vary depending on the cut, sex, age, and breed of the meat evaluated (Jayasena et al., 2015a; Jung et al., 2013). Several previous studies have reported similar differences in the metabolites of these two meat portions (Jayasena et al., 2014a; Jayasena et al., 2015b; Jung et al., 2013). Breast meat primarily comprises type IIB muscle fibers (fast-twitch glycolytic white fiber) with relatively less type IIA fibers (fast-twitch oxidative glycolytic white fiber), while thigh meat contains high levels of type I (slow-twitch oxidative red fiber) and IIA fibers (Jaturasitha et al., 2008). Type II muscle is known to rely considerably on glycolytic metabolism for its energy supply because these muscles have fewer mitochondria than those observed in type I muscles (Booth and Thomason, 1991). This indicates that carnosine accumulation occurs more frequently in type I muscle fibers compared to that in type II muscle fibers (Jayasena et al., 2015b), thereby explaining the differences in its concentration in breast and thigh tissues. Taken together, these data suggest that KNC-D breast meat, which has more taste-active and bioactive compounds, is the best candidate to replace commercial KNC-H and WSB breeds for Samgye.
Multivariate analysis can easily identify the differences in the metabolic characteristics of different samples (Ergon, 2004). Our data were classified using PCA and PLS-DA (Fig. 2). The PCA score plots show that meat portion (breast and thigh) and breed (KNCs and WSB) can be clearly distinguished by PC 1 (69.3%) and PC 3 (6.8%), respectively (Fig. 2a). This was also true for the PLS-DA score plots where components 1 and 2 allowed for easy differentiation (R2=0.945; Q2=0.901; data not shown). Additionally, groups were easily distinguished by meat portion and breeds when using both the cumulative explained variation (R2) and predictive ability (Q2) values. Most of the metabolites (indicated by yellow dots; VIP scores>1) on the loading plots could be observed in one of two extremes in PC1, which indicated that more important variables correlated substantially with the meat portion rather than the breed. This may primarily be the result of the major metabolic differences between type I and type II muscle fibers and the distinct differences in their distribution between thigh and breast meat (Jayasena et al., 2015b; Jung et al., 2013).
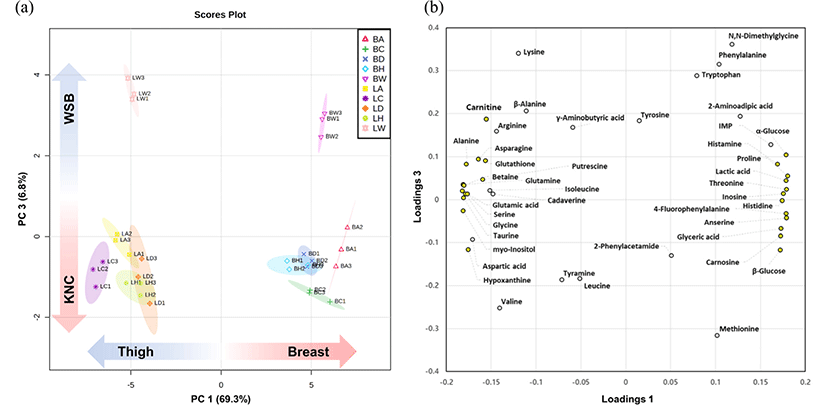
Samples can be arranged in a clustered heatmap based on the similarities in their metabolic information (Škuta et al., 2014). Here, our clustered heatmap analysis (Fig. 3) showed that whole metabolic profiles could be used to indicate breed even following hierarchical analysis by meat portion. WSB samples were completely distinct from the KNC samples, suggesting that these chickens demonstrated a markedly different metabolism from the KNCs. Different meat types may have different metabolomes, resulting from different postmortem metabolic rates (Ryu and Kim, 2005), suggesting that this metabolic information may be used to differentiate meat samples for meat authenticity using machine learning algorithms, such as SVM and random forest classification, which can also be used to elucidate metabolic differences without performing quantitative analysis (Winning et al., 2008; Xia and Wishart, 2016). Based on the PCA and clustered heatmap analysis, multivariate ROC curve analysis was then used to validate the discriminatory value of these differences between KNCs and WSB in both meat types.
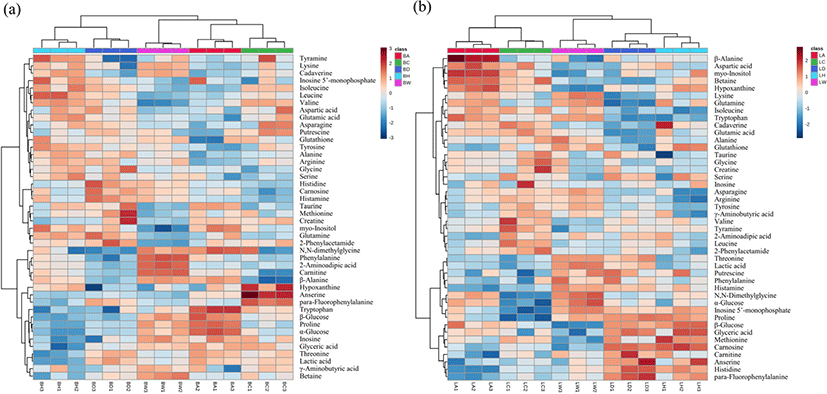
Multivariate ROC curve analysis using linear SVM algorithms was evaluated for optimal model selection (Fig. 4), and the area under the ROC curve (AUC) was shown to be highest when the model used 10 features with good confidence interval values. This model demonstrated excellent overall discriminatory ability (>0.90) in both breast and thigh meat (Muller et al., 2005). Additionally, this model did not exhibit class probability errors and every sample was correctly classified (type I and type II errors) in both meat types (Fig. 4b and 4e). The 10 features selected for the breast meat were carnitine, 2-aminoadipic acid, phenylalanine, β-alanine, myo-inositol, betaine, valine, cadaverine, lysine, and N,N-dimethylglycine (Table 2). Carnitine is synthesized from lysine and methionine (Kim et al., 2020b), and betaine is converted into dimethylglycine (Friesen et al., 2007). Likewise, other metabolic pathways may cause slight differences in the profiles of KNC and WSB breast meat.
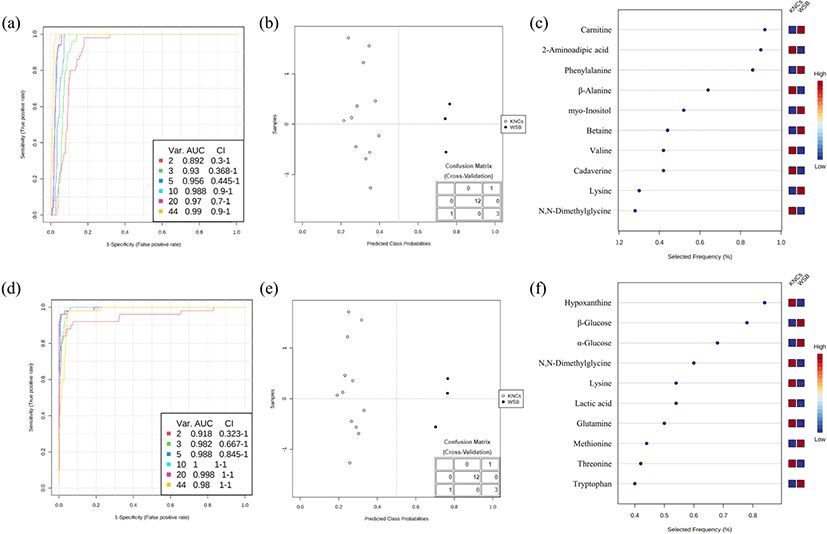
N,N-dimethylglycine and lysine levels were lower in KNC thigh meat than those in WSB thigh meat (Table 2), while hypoxanthine content was higher in KNC samples compared to that in WSB samples, thereby presenting with lower IMP and rapid accumulation. In both the breast and thigh meat, WSB samples presented with higher amino acid content than the KNCs and this high concentration of free amino acids might be associated with the higher growth rate; this might promote faster muscle development in these chickens (Palma et al., 2016). Few ranked metabolites such as 2-aminoadipic acid and myo-inositol have rarely been reported in poultry meat. Additionally, metabolites undergo proteolytic enzyme cleavage and nucleotide degradation during the transition from muscle to meat and metabolic concentrations and end products can also change depending on the sex, species, and age (Jayasena et al., 2014b; Jung et al., 2013; Kim et al., 2020a; Ryu and Kim, 2005), suggesting that further analysis may be necessary to identify critical metabolic properties in these animals. However, despite this, our data were in agreement with those reported in previous studies which suggested that the combination of qNMR and multivariate analysis could elucidate metabolic characteristics of samples in the absence of numerical quantification (Kim et al., 2020a; Kim et al., 2020b; Winning et al., 2008).
Conclusions
Our results show that newly developed KNC-D chickens present with higher anserine, creatine, carnosine, and IMP contents in the breast tissues than those observed in the commercial KNC and WSB chicken breeds. Meat portion and breed were also clearly distinguishable using PCA and hierarchical analysis based on the 2D HSQC analysis. Moreover, ROC analysis was useful for distinguishing between different breeds. Based on these results, we suggest that a metabolomics approach to identify breeds based on 2D HSQC analysis demonstrates superior performance to the conventional quality assessment tools and can differentiate between breeds and samples when used as part of a multivariate analysis. However, further analysis is warranted to determine the exact biomarker necessary to distinguish between each breed.