Introduction
In recent years, donkey meat has been increasingly favored because of its high protein, low fat and tender meat characteristics (Cocolin et al., 2013; Polidori et al., 2008). However, these characteristics provide a rich substrate for the growth of microorganisms, which leads to the deterioration of fresh donkey meat. Refrigerated storage is a common method of inhibiting microbial growth among varieties of preservation techniques. Numerous studies on refrigerated storage of meats have been reported (Ercolini et al., 2011; Giannuzzi et al., 1998; Marino et al., 2015; Wang et al., 2019). However, little information on refrigerated donkey meat is available. Therefore, studying the microbial community succession in donkey meat during refrigerated storage is important for preventing meat putrefaction and extending the shelf life.
Traditional cultivation methods and molecular techniques, such as real-time quantitative PCR (qRT-PCR), denaturing gradient gel electrophoresis (DGGE) and temperature gradient gel electrophoresis (TGGE), were used to investigate the composition of microbial communities in meat (Ercolini et al., 2013; Van Reckem et al., 2021). However, the information obtained from these methods was limited, and could not reflect the changes of microorganism comprehensively (You et al., 2016). Recently, Illumina NOVA sequencing technology based on the 16S rDNA has been used to analyze the composition of microorganisms (Cao et al., 2021; Pfaffl and Riedmaier-Sprenzel, 2018). The result showed that white muscles were dominated by Aeromonas in the early storage period, and dark muscles were dominated by Aeromonas, Pseudomonas and Lactococcus. And Pseudomonas was the dominant species in the two kinds of different color muscles after corruption (Li et al., 2018). The microbial population changed significantly during storage, among which Micrococcaceae and Flavobacteriaceae occupied the absolute dominance in the later period (Zhao et al., 2015). Nevertheless, the application of this method to refrigerated donkey meat has not been reported. In this study, bacterial community dynamics were investigated in donkey meat during refrigerated storage by Illumina NOVA sequencing technology. The volatile base nitrogen (TVB-N) was measured to evaluate the degradation level of protein. The purpose of this study was to reveal the relationship between spoilage and bacterial community composition, identify the metabolic function of donkey meat during refrigerated storage, and explain different spoilage characteristics from the perspective of microbial metabolic function.
Materials and Methods
The sampling method was referred to Zhang with slightly modificated (Zhang et al., 2018). Fresh donkey meat from the longissimus of 2-year-old male Dezhou donkey were purchased from Dong-E-E-Jiao (Liaocheng, China), then stored in a sterile crisper at 4°C which covered the seal with sterilized polyethylene plastic wrap immediately. Samples of refrigerated donkey meat were taken from the same fillet every 2 days for microbial analysis and quality analysis. The experiment was set in four parallel settings.
The TVB-N content was measured with 10 g of samples according to the national standard method (GB/T 5009.22-2016). The value expressed as mg TVB-N per 100 g of meat and was measured in triplicate.
Extraction of bacterial DNA followed the procedure of Xiao with some modifications (Xiao et al., 2013). Twenty-five grams of donkey meat sample was cut up and mixed with 50 mL sterile saline, and then centrifuged at 3,000×g for 10 min. Then the supernatant was centrifuged again at 12,000×g for 10 min. After that, the supernatant was removed and the precipitate was washed with 1.0 mL sterile water. DNA extraction kit (Qiagen 51604) was used to extract bacterial DNA from the precipitation mixture. Three parallel DNA from the same donkey meat sample and storage conditions were mixed together. The DNA extraction was stored at −60°C for further analysis.
PCR amplicons were obtained from the V3-V4 regions of the 16S rRNA gene and analyzed for bacterial diversity on the Illumina NovaSeq PE250 platform. Sequencing primers for PCR amplification were 515F (GTGCCAGCMGCCGC GGTAA) and 806R (GGACTACHVGGGTWTCTAAT) with the following protocol: 98°C for 5 min, followed by 27 cycles at 98°C for 30 s, 56°C for 30 s, and 68°C for 60 s and a final extension at 72°C for 7 min. The amplified PCR products were detected by 2% agarose gel electrophoresis and purified using the Gene JET Genomic DNA Purification Kit. Each sample was analyzed in triplicate.
QuantiFluor-ST (Promega, Madison, WI, USA) was used to quantify the PCR products, and the TruSeq® DNA PCR-Free Sample Preparation Kit was used to build sequencing libraries. The library was paired-end sequenced by using the Illumina NovaSeq6000 system (Illumina, San Diego, CA, USA). Paired and clean dates were merged using FLASH (V1.2.7, http://ccb.jhu.edu/software/FLASH/) and filtered by Qiimer (V1.9.1, http://qiime.org/scripts/split_libraries_fastq.html) with a removal of syncyte sequence (https://github.com/torognes/vsearch/) (Bokulich et al., 2013; Caporaso et al., 2010; Magoč et al., 2011). Effective tags with 97% similarity were clustered into OTUs cluster using UPARSE (v7.0.1001, http://www.drive5.com/uparse/) (Haas et al., 2011). Based on Mothur (v1.39.1, https://www.mothur.org/) and SILVA Database (https://www.arb-silva.de/), the representative sequences from each OTU were annotated as species (confidence interval 0.8–1.0), to obtain the microbial composition of different samples at various taxonomic levels (Edgar et al., 2013). QIIME v1.9.1 was used to measure bacterial richness and diversity using alpha-diversity indexes such as Good’s coverage, Chao 1, ACE, Shannon index, and Simpson's index (Kemp et al., 2004). Tukey test was used to determine the significance of differences in Alpha diversity index. To analyze the difference in bacterial community composition of samples, beta-diversity analysis including principe component analysis (PCA), multi response permutation procedure (MRPP), and linear discriminant analysis effect size (LEFSe) was performed. The differential bacteria were defined by the conditions (LEFSE, p>0.05 and LDA>2, Metastats, p<0.05 and Q<0.1 with relative abundance>10−3) (Ji et al., 2019). Vegan software in R software (v.3.1.1) was used to analyze non-metric multidimensional scaling (NMDS) two-dimensional graph at non-metric scale (Liu et al., 2019).
Results and Discussion
A total of 1,058,807 high-quality valid sequences were obtained through quality control and integration of the original sequences of each sample (Table 1). The length of these sequences ranged from 400 to 450 bp on average. A minimum similarity threshold of 97% was used to produce 221–869 OTUs per sample. The ACE and Chao1 indexes followed the same pattern as the OUT numbers, decreasing first and then rising, which was consistent with previous findings (Zheng et al., 2020). The results showed that the Good's coverage rate of all samples were above 99.5%, which indicated that nearly all bacteria in the samples could be identified at the present sequencing level. At the end of the storage time, the values of Chao 1, ACE, Shannon and Simpson indexes (d7) were significantly lower than the initial values (d0) (p<0.05), suggested that a subset of bacteria became dominant in samples, which are similar to the variation of microbial community Alpha diversity during the storage of chilled pork (Zhou et al., 2020).
A total of 32 phyla were identified in the sequencing analysis, including Proteobacteria, Firmicutes, Bacteroidetes, Acidobacteria, Actinobacteria, and Cyanobacteria (Fig. 1A). Proteobacteria and Firmicutes were the major phyla during storage, accounting for 84% of all OTUs, which were consistent with poultry, livestock and aquatic products (Hou et al., 2021; Li et al., 2018; Li et al., 2019a; Saewa et al., 2021), which indicated that there is no distinction between species. With the extension of storage time, the proportion of Proteobacteria in each sample rose from 74.10% to 97.10%, and the proportion of Firmicutes dropped from 10.20% to 1.40%. There seemed to be no significant difference in microbial communities in different storage times with Proteobacteria and Firmicutes predominanted. These results indicated that Proteobacteria and Firmicutes might be the main bacteria phyla in the process of meat spoilage in cold storage, which played an important role in the quality change.
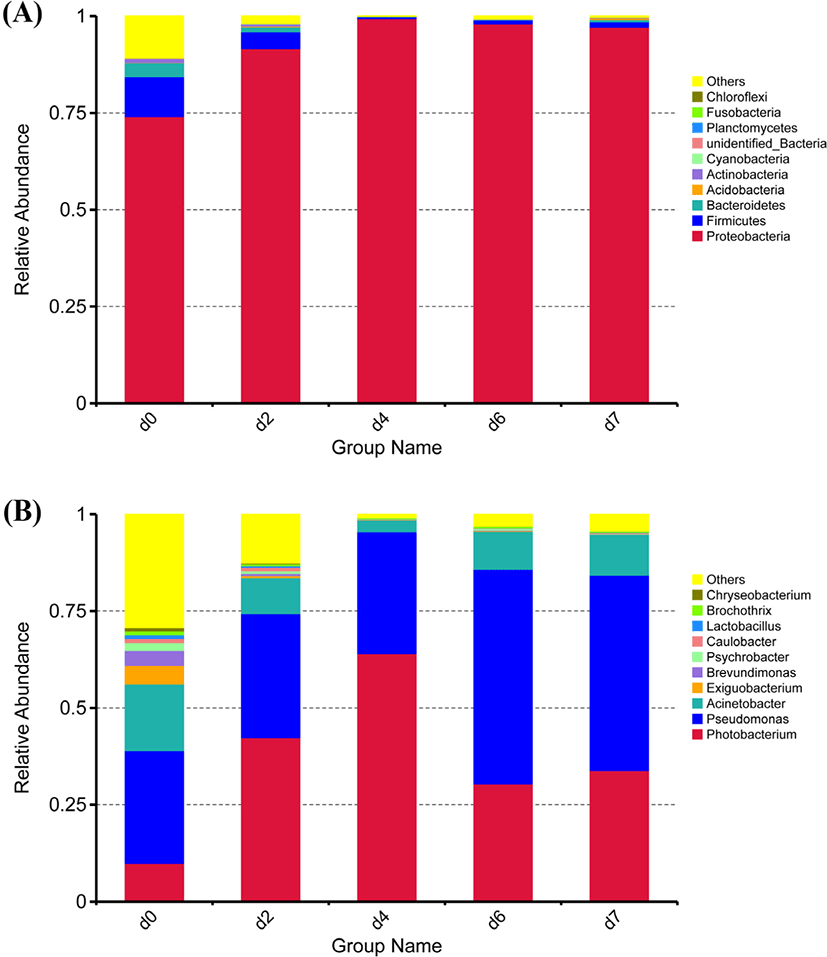
Bacterial community dynamics were evaluated based on changes in relative abundance at genus level during storage (Fig. 1B). A total of 527 bacteria genera were identified by the sequencing analysis, including Pseudomonas, Photobacterium, Acinetobacter, Exiguobacterium, and Brevundimonas. As the storage time increased, Photobacterium showed a trend of decreasing first and then increasing, but Pseudomonas was the dominant bacteria which are consistent with the experimental results of Yang (Yang et al., 2018). Related research reports showed that Pseudomonas were common spoilage bacterias in the process of low temperature storage of meat products, with strong production ammonia and other putrefaction products (Shaw and Latty, 1982). In meat storage, Pseudomonas could become the dominant genus mainly because it could decompose and utilize protein (Doulgeraki and Nychas, 2013).
A genus-level clustering heatmap based on the top 35 genera in relative abundance was constructed to analyze and compare the composition and dynamic changes of microbial communities in different samples (Fig. 2). The heatmap showed that the relative abundance of different bacterial communities in each time period changed greatly. The redder and the greener illustrated the higher and the lower relative abundance, respectively. Due to the abundance of nutrients, microorganisms multiply rapidly and their diversity increased at the beginning of storage. However, it showed a certain degree of stability between 4 and 7 days. As the storage time increased, Photobacterium showed a trend of decreasing, which became the dominant bacterium (d4). At the end of the storage, Pseudomonas and Acinetobacter increased, then became the dominant bacterium with Photobacterium. The relative abundance of Acidisoma, Granulicella, and Shewanella increases as well. There was a significant difference in the relative abundance of each genus between the early storage and the late storage in the heatmap. Shewanella mostly came from the spoilage of fish and shrimp meat (Lund et al., 2000). Acidisoma had a relatively high relative abundance, but the relative abundance was only 0.2% on the 7th day, so it does not contribute much to the deterioration of donkey meat. Therefore, it is not a kind of typical metamorphic bacteria in donkey meat. Acidisoma belongs to Alpha proteobacteria that were metabolized by chemical energy (Belova et al., 2009). The result indicated that it could be metabolized by carbohydrates and organic acids. The increase in relative abundance on the 7th day was probably due to the hydrolysis of fat in donkey meat.
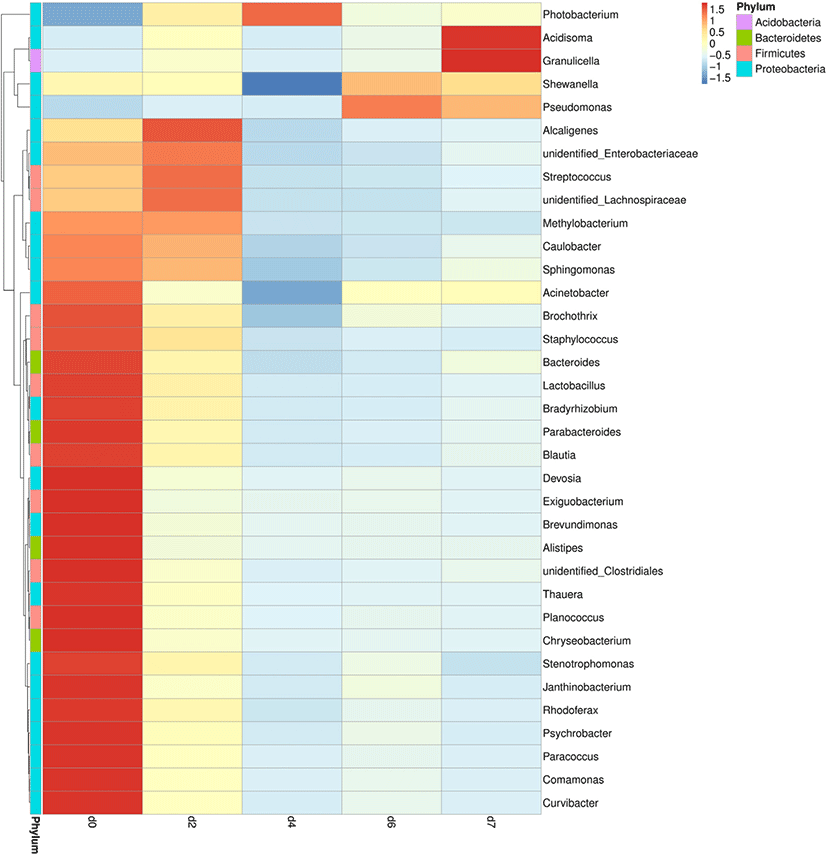
In summary, the study found that with the prolong of storage time, Pseudomonas, Photobacterium and Acinetobacter were the characteristic spoilage bacteria in donkey meat. The relative abundance of dominant bacteria in refrigerated donkey meat was dynamically changing, but the dominant bacteria category was not changed.
NMDS two-dimensional graph at non-metric scale was constructed to analyze the differences of bacterial communities during storage times (Fig. 3). The closer the distance between the coordinate axes, the higher the composition similarity between the samples. In the early storage (d0 and d2), the aggregation was the highest, which indicated that the bacterial community structure of the samples in the early stage of storage was relatively similar. With the prolong of storage time, the composition of microorganisms in the samples increased. There were significant differences in community structure between early storage (d0 and d2) and late storage (d6 and d7) (p<0.05). However, there was no significant difference in early and late storage. Similar conclusions were supported from analysis of chilled beef from different sources (Säde et al., 2017). This indicated that there was not enough superiority between microbiota in the early stage, but the microbiota in the later stage were gradually replaced by the dominant bacteria, which may be related to the influence of pH and secondary metabolites on the flora structure (Ingram and Dainty, 1971).
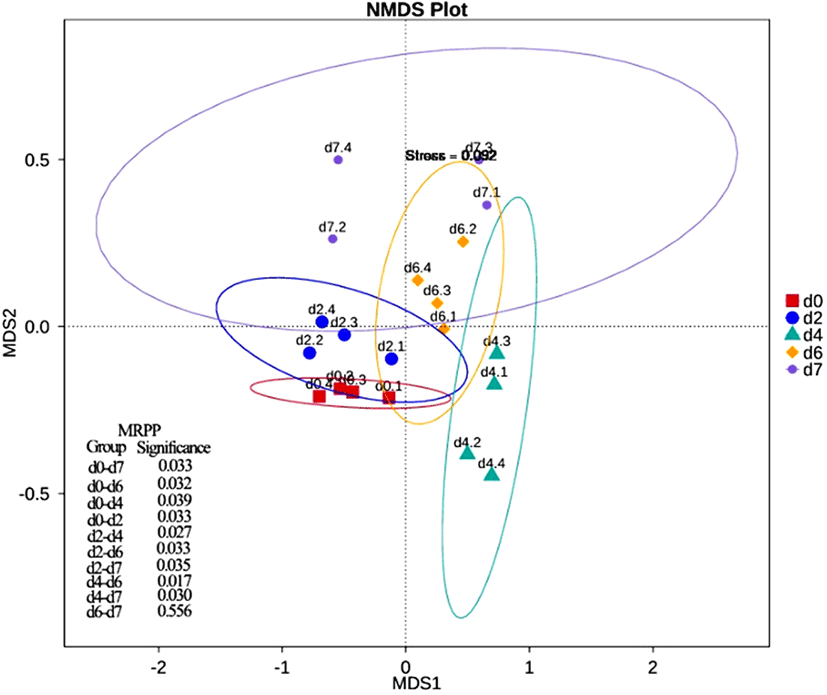
Bacteria with significant differences (relative abundance>10−3) were counted to analyze the differences of bacterial communities at different storage times (Table 2). In order to further explore the dominant microbial species, which caused the deterioration of donkey meat, the bacteria genera with relative abundance>10−3 were selected as the research object (Li et al., 2019b). The significant differences in the storage time were shown in Table 2. The d0 of Photobacterium spp. was extremely significantly lower than other times (p<0.01), the fourth peak reached the highest, and d4 was extremely significantly higher than d6 or d7 (p<0.01). Pseudomonas d0 was extremely significantly lower than d6 or d7 (p<0.01), d6 was extremely significantly higher than d2 or d4 (p<0.01), the difference was not significant at other times. The study of Wang also obtained the similar results (Wang et al., 2014). Acinetobacter was extremely significantly higher in d0 than other times (p<0.01). However, it was extremely significant lower in d4 than other times d4 (p<0.01), Brevundimonas and Psychrobacterium were extremely noteworthy significant higher in d0 than d4, d6, and d7 (p<0.01). Some studies reported that Pseudomonas was the main microorganism that caused meat spoilage under aerobic cold storage (Parlapani et al., 2015; Stellato et al., 2017; Wang et al., 2021). At the same time, Acinetobacter, Brevundimonas, and Psychrobacter cannot form influential competition with them (Mohareb et al., 2015; Rodríguez-Calleja et al., 2005). In this study, the increased number of Photobacterium and Pseudomonas showed that the microbial community had undergone tremendous changes during storage. It may be very important to control the growth of Photobacterium and Pseudomonas for prolonging the shelf-life of donkey meat in a specific storage period.
The number of microbial metabolic pathways and the relative abundance of metabolic pathways determine the speed and extent of the deterioration of meat products. The prediction of homologous protein clusters of functional genes by using the PICRUSt was used to analyze the changes of microbial metabolic function in refrigerated donkey meat in different storage times (Fig. 4, Fig. 5). The screening identified 10 pathways related to metabolism. Except for fat metabolism, all metabolic pathways were significantly different at the end of storage (d7) and the beginning of storage (d0) (p<0.05). During the entire storage process, the relative abundance of amino acid metabolism and carbohydrate metabolism accounted for a high proportion, which was consistent with previous studies (Li et al., 2019a). Donkey meat had the highest proportion of amino acid metabolism in bacterial flora, which might be due to the high protein content of donkey meat. Stellato et al. (2016) found that the proportion of amino acid metabolism in each metabolic function was the main function in pork and beef. Metabolic pathways were gathered in 2 clusters together with late storage (d6 and d7) and or mid-term (d2 and d4), which might be caused by the bacterial diversity decreases in late cold storage period. The result also found that the relative abundance of amino acid and fat metabolism increased during storage prolonged. Ercolini et al. (2010) reported that Pseudomonas had strong lipid and protein hydrolysis, which had the highest relative abundance in the late storage period. Furthermore, the relationship between different bacterial genera and metabolic pathways were also analyzed. Glucose and lactic acid were used up by Pseudomonas firstly, and then the protein started to be consumed (Nychas et al., 2008). Combined with the Fig. 6, Pseudomonas was significantly positively correlated with amino acid metabolism, which was consisted with the increase of relative abundance (Fig. 1B) and amino acid metabolism (Fig. 5) of Pseudomonas in late period (d6 and d7).
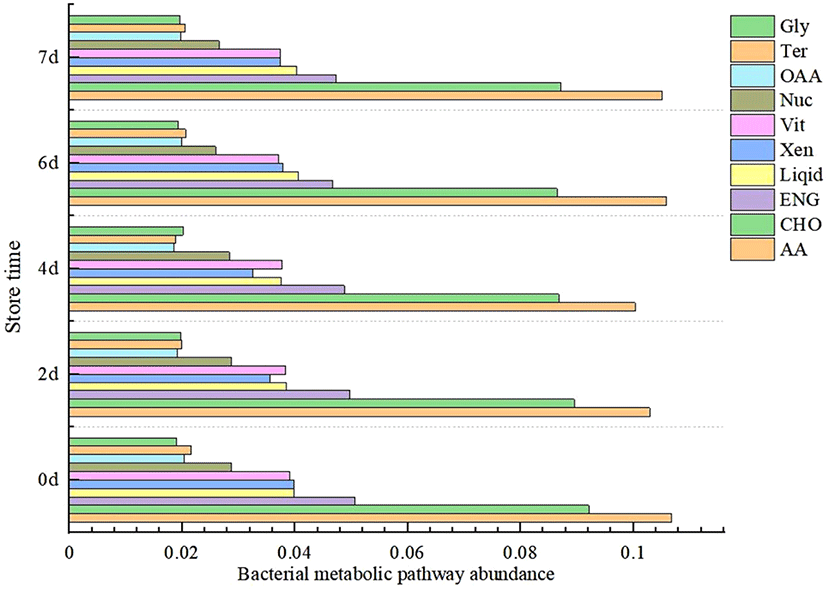
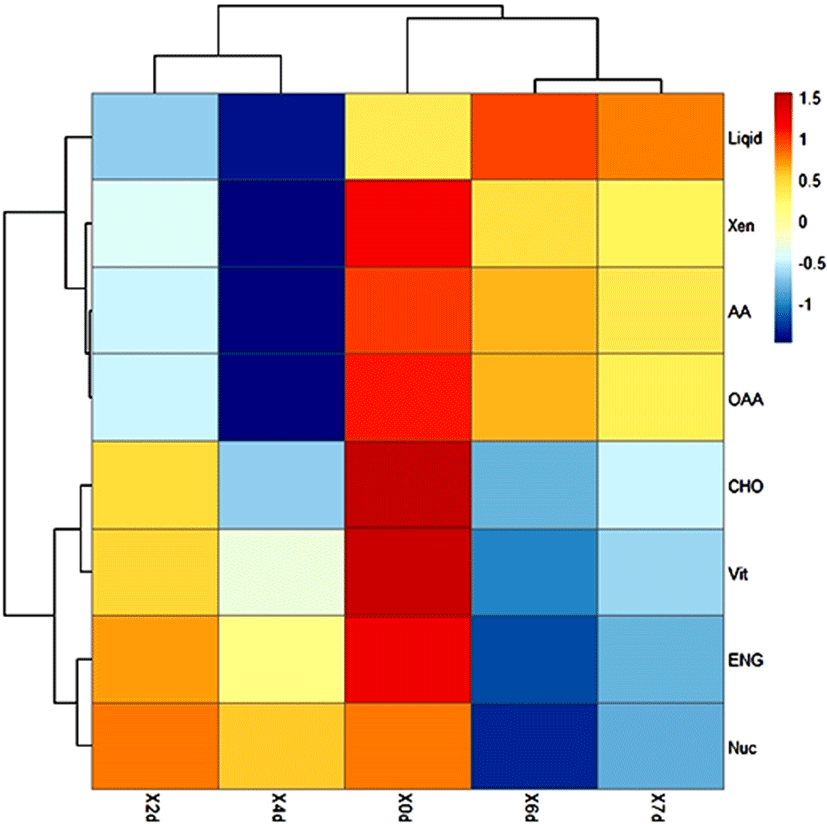
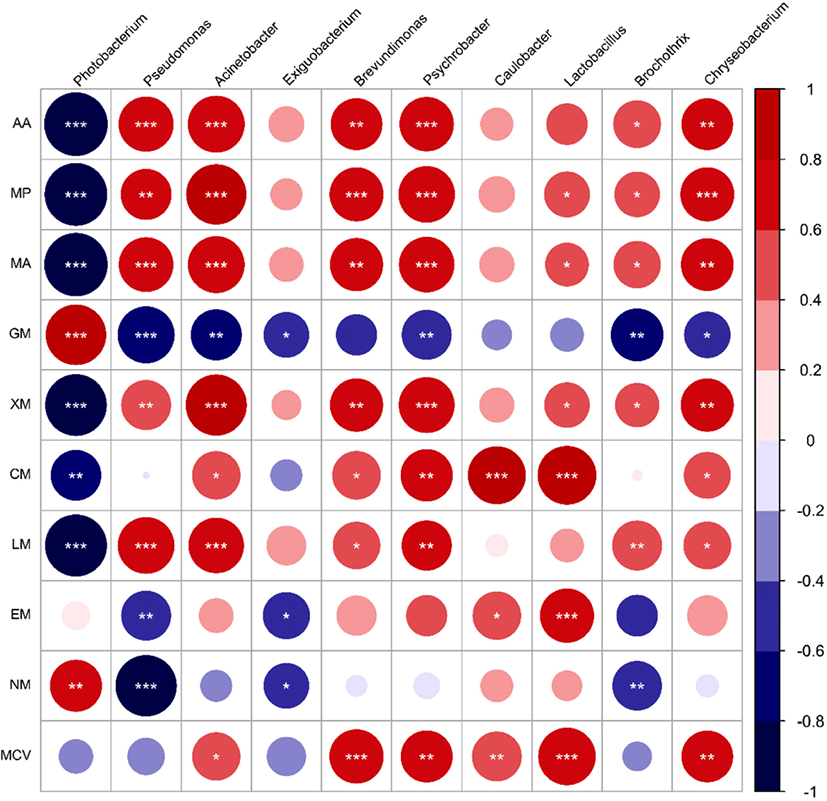
The volatile base nitrogen (TVB-N) is the total amount of nitrogen extracted from the aqueous extract of meat or meat products (under alkaline conditions), which produced by the effects of decarboxylation and deamination caused by microbial reproduction in meat (Lee et al., 2018). It is an important index to evaluate the freshness of meat and meat products. And it is stipulated that TVB-N of fresh (frozen) livestock and poultry products should be less than 15 mg/100 g in China.
The TVB-N content was detected to analyze the changes of refrigerated donkey meat in storage period (Table 3). The results indicated that the content of TVB-N increased significantly with the storage time extend (p<0.05). The donkey meat was edible within 4 days, after that began to rot and deteriorate. After 7 days of storage, the content of TVB-N reached 18.32 mg/100 g, which was far above edible standards (Maršićlučić et al., 2008). TVB-N with the extension of time maybe was caused by nitrogen-containing substances degradation, such as proteins into amines and ammonia substances, which caused by endogenous enzymes and bacteria (Duan et al., 2019; Kulawik et al., 2013). At the early stage of storage, the content of TVB-N was 7.16 mg/100 g, different from that of pork and beef, which might be related to different meat varieties (Cai et al., 2011; Li et al., 2019c).
Store time (d) | TVB-N (mg/100 g) |
---|---|
0 | 7.16±0.29h |
1 | 8.28±0.48g |
2 | 9.67±0.50f |
3 | 10.95±0.95e |
4 | 13.89±0.45d |
5 | 15.14±1.26c |
6 | 17.27±1.04b |
7 | 18.32±1.73a |
Pearson correlation analysis was performed on the relative abundance of TOP 10 bacteria genus, and TVB-N content using SPSS in different storage times (Table 4). A total of 7 genera bacterial were screened which were significantly correlated with TVB-N (p<0.05). There was a significant positive correlation between Pseudomonas and TVB-N content. Pseudomonas might play an important role in the production of TVB-N during cryopreservation of donkey meat (Liang et al., 2021; Na et al., 2018).
Conclusion
This study was conducted to reveal the microbial community succession and protein hydrolysis of donkey meat during refrigerated storage with Illumina NOVA sequencing technology. A total of 527 bacteria genera were identified by the sequencing analysis, and Photobacterium and Pseudomonas were the dominated microorganisms at late storage. The prediction of homologous protein clusters of functional genes predicted that the carbohydrate metabolism and amino metabolism were the major metabolism in refrigerated donkey meat in different storage times. Pearson correlation analysis indicated that Pseudomonas might play an important role in the production of TVB-N during refrigerated storage of donkey meat. Our study deepened the understanding of the microbial decay mechanism of refrigerated donkey meat, and provided theoretical support for the development of donkey meat preservation technology in the later period.