Introduction
Meat and meat products are popular among consumers because they are both tasty and nutritious. The quality and taste of meat are the results of various complex factors, with color, water-holding capacity (WHC), tenderness, and flavor being their primary determinants (Joo et al., 2013). In general, meat quality and taste characteristics vary depending on the livestock species, breed, gender and slaughter period etc. Even the same breed can have different quality and taste characteristics depending on individual muscles (Kumar et al., 2021; Lee et al., 2023b; Won et al., 2023). Feeding management (Hossain et al., 2023; Kim et al., 2023a), slaughter weight, slaughtering processes (Hwang et al., 2022; Oh et al., 2022), and processing methods (Ismail et al., 2022; Lee et al., 2023a) can also influence meat quality and taste characteristics. Therefore, exploring how primary determinants of meat quality change according to these factors and what mechanisms are involved can increase the possibility of high-quality meat production. Although there is an urgent need to understand the molecular mechanisms responsible for biochemical or biological changes in meat quality, methods for measuring and evaluating these biochemical and/or biological changes objectively are lacking unfortunately. Hence, it is important to understand the technical principle of approaches used to analyze the quality and taste of meat, to improve the accuracy to better reflect changes in quality and taste, and to identify the molecular mechanisms responsible for high-quality and delicious meat (Gagaoua et al., 2021; Kim et al., 2023b).
Recent advances in high-throughput sequencing technology and high-resolution mass spectrometry are enabling a comprehensive and systematic understanding of meat quality and taste characteristics at the molecular level through intensive multi-omics studies (Ramanathan et al., 2020). Commonly used omics techniques related to the quality and taste of meat are mainly genomics, including transcriptomics, proteomics, and metabolomics. Multi-omics techniques integrating these individual omics are very useful for exploring related genes, proteins, and metabolites that can improve meat quality and taste (Ramanathan et al., 2020). Differentially expressed biomolecules identified using omics can not only be used as biomarkers to predict meat quality, but also can be applied to find interactions at the molecular level. For these reasons, a number of recent studies using multi-omics technologies have successfully explored impacts of various physiological processes on meat quality during meat production (Kim and Kim, 2021; Purslow et al., 2021; Wu et al., 2020). Moreover, multi-omics technologies have been used to investigate biomarkers and biological processes related to meat quality. However, there is still a lack of reviews on multi-omics technologies in relation to meat quality and taste characteristics.
In this respect, this review introduces technological principles of genomics, transcriptomics, proteomics, metabolomics, and multi-omics in order. Firstly, an overview technology of genomics related to meat quality is introduced, focusing on the structure and function of genes that can affect meat quality traits. Next, technical principles of transcriptomics and its application in meat research are introduced, focusing on effects of genes of various breeds and feeding management on fat deposition and muscle fiber development. Principles of proteomics technologies and related application in meat with effects of pre- and post-slaughter factors on meat at the proteomic level are then summarized. In addition, major principles of metabolomics technologies and their applications in meat are reviewed in relation to metabolites that can cause changes in flavor and tenderness are summarized. Finally, this paper introduces applications of multi-omics in meat research, focusing on integrated methodological studies of biological systems. Future perspectives of multi-omics are also discussed.
Genomics Approaches to Improve Meat Quality
Genomics is the study of all genes of animals or individuals. Genomics analysis provides useful information about intragenomic interactions in the genome. Genomics can be used to identify particular markers, genomic regions, candidate genes associated with economically significant trait and evaluate an individual’s breeding value (Goh et al., 2018; Kim et al., 2023c; Lee et al., 2023b; Raschia et al., 2018; Won et al., 2023). It can also be used to study the structure and function of genes that affect meat quality traits. The development of sequencing tools has enabled the expansion of functional or expressive genomics as knowledge about genomes builds up. Advancement in functional genomics research is enabled by the ability to simultaneously analyze hundreds or thousands of genetic polymorphisms [single-nucleotide polymorphisms (SNPs) with chips], transcripts (transcriptomics), proteins (proteomics), and metabolites (metabolomics) with dedicated arrays or specific tools. Over the past few decades, these tools have been implemented to identify genes, proteins, and/or metabolites that are related to meat quality traits or taste characteristics beyond the production of meat (D’Alessandro et al., 2012; Kim and Kim, 2021; Picard et al., 2015).
The ultimate objective of genomics for meat science and industry is to identify meat quality biomarkers that can be quantified in the early postmortem period of carcass or alive animals in order to produce and distribute good quality meat during meat processing or distribution. Quantification of these biomarkers can predict meat quality from birth in the case of SNP biomarkers. Other biomarkers such as genes, proteins, and metabolites can be used to predict the quality level of meat from livestock growth to slaughter. Although these genomic evaluations are typically expensive as they are performed invasively within days of slaughter or even in sufficiently aged meat samples, they provide substantial and valuable information. For example, the use of expression biomarkers can allow us to understand interactions between genetic and environmental factors that contribute to the development of complex phenotypes such as meat color, WHC, tenderness, marbling, umami intensity, and so on. This knowledge can be used to produce meat of better quality and enhanced its palatability. Indeed, over the past few years, these technologies have been implemented in most meat production systems (Hollung et al., 2007; Lim et al., 2009; Park et al., 2021; Picard et al., 2015).
A practical application of genomics is to develop biomarkers for meat quality traits by sampling from either live animals or postmortem carcasses. One of the representative and practical implications is the identification of RYR1 gene mutation (Fujii et al., 1991) and associated effects in the development of PSE (pale, soft, exudative) condition. However, studying one gene may not provide meaningful information because each meat quality trait is polygenic and influenced by various genes. One of the representative methods used in genomics is identifying variations in DNA sequences between different animals followed by determination of how they correlate with each meat quality trait. The most common type of genetic variation is SNP, which can help us predict quality variations such as muscle pH, tenderness, marbling, and so on (Cho et al., 2015; Kim and Kim, 2021; Lim et al., 2009).
Besides successful implementation of the human genome project, livestock has also paved the way to sequence the entire genome of a species. Genome-wide association studies of livestock have enabled identification of genes related to meat quality traits (Cho et al., 2023; Park et al., 2021). Each study can look at thousands of SNPs at the same time and pinpoint genes that can contribute to a specific meat quality defect. These genomic analyses involve enzyme digestion to cut DNA extracted from meat samples into smaller strands. These small strands are later incubated with primers to promote binding to complementary bases. If ChIP-sequencing (chromatin immunoprecipitation) is used, a large amount of data can be generated as multiple genes can be identified in a single run. Computational software can be used to analyze such big data of gene sequences to identify several genes related to a meat quality trait (Goh et al., 2018). However, some genes identified by ChIP-sequencing might not be functional. Hence, interest in mRNA quantification is recently increased. In other words, many studies are being conducted to quantify mRNA (transcriptomics) to more accurately understand changes in meat quality and palatability of meat (Cho et al., 2023; Kim and Kim, 2021; Kim et al., 2023d; Won et al., 2023).
Transcriptomics Techniques and Application for Meat Quality
Transcriptomics intensively investigates the transcription and regulation of mRNA and ncRNA in cells or tissues under specific conditions. It is known that ncRNA regulates initiation and post-transcriptional modification of mRNA transcription. It can be classified into miRNA, IncRNA, and circRNA. One of the key functions of lncRNA is to modulate gene expression through diverse mechanisms (Park and Kim, 2023). RNA is closely related to the expression of meat quality traits because it is involved in organs, muscles, muscle growth and development, muscle fiber type transformation, and intramuscular fat deposition (Jung et al., 2019; Morenikeji et al., 2020; Wang et al., 2019a). Therefore, it is very important to explore transcriptomics of muscle growth regulatory factors such as myostatin and insulin-like growth factors that play a role in regulating muscle growth. In other words, transcriptomics, which detects spatiotemporal specific expression of genes under different states, is useful for explaining the action mechanism responsible for changes in meat quality.
Transcriptomic technique has evolved through three technological stages: reverse transcription-polymerase chain reaction (RT-PCR), gene microarray, and RNA-seq. RT-PCR converts RNA to cDNA, uses cDNA as a template for amplification, and detects the expression of target gene after amplification based on fluorescence in the reaction tube (Heller et al., 2019; Kang et al., 2023). Gene microarray technology, on the other hand, is based on the principle of complementary base pairing. It uses inkjet technology or lithography mask to fix cDNA on the matrix to measure RNA abundance (Castillo et al., 2017). The abundance of RNA is determined by fluorescence intensity before and after the reaction of test sample with cDNA on the matrix (Goh et al., 2018; von der Haar et al., 2017). However, gene microarray has several disadvantages. In the case of genes with high RNA abundance, there is a threshold for microarray technology. In the case of genes with low expression abundance, fluorescence signals can be overwritten, making detection difficult. Moreover, it is difficult to detect unknown splicing variations when the background noise is high (Bolón-Canedo et al., 2019). RNA-seq is the state-of-the-art technology introduced to overcome these limitations. This method enables the detection of transcripts from organisms with unknown genomic sequences and facilitates precise RNA quantification (Kang et al., 2022). RNA-seq was first introduced in 2008. Progressive sequencing technology has been developed (Goh et al., 2018; Jazayeri et al., 2015; Park and Kim, 2023). The first-generation sequencing technology is represented by Sanger, which has a low throughput. Although second-generation sequencing has the advantage of high throughput sequencing, short fragments are preferred due to variations among different strands during the replication process. Third-generation sequencing techniques achieved ultra-long read lengths by connecting sequenced RNA molecules directly to loops. However, its accuracy of sequencing needs to be improved. Consequently, second-generation sequencing is currently the most widely used one due to its high throughput advantage. Among second-generation sequencing tools, advantages of NGS are more obvious than those of pyrosequencing (Sim et al., 2022).
Recently, a series of studies have been conducted to explore genes with the potential to produce better meat quality using transcriptomics, particularly those involved in the proportion and composition of intramuscular fat in longissimus dorsi muscles. Content and fatty acid composition of intramuscular fat affecting marbling are important factors for evaluating meat quality, which can directly affect not only meat quality traits (such as meat color, tenderness, and juiciness), but also meat taste characteristics (Hwang et al., 2020a; Joo et al., 2013). Transcriptomic analysis has revealed genes involved in fatty acid synthesis, triglyceride catabolism, gluconeogenesis, ion transport, transporter activity, and so on (Kumar et al., 2021). For example, the FASN gene can catalyze the synthesis of long-chain fatty acids, while FABP3 gene has been found to be primarily involved in cell metabolism and transport of long chain fatty acids (Piórkowska et al., 2020). The SCD gene encoding stearoyl-CoA desaturase has also been found to be able to convert trans fatty acids (C18:1) to conjugated fatty acids (Czerniawska-Piątkowska et al., 2021). In addition, genes such as GPAT, AGPAT, and DGAT have been revealed to be related to the formation of intramuscular fat accumulating between muscle fibers and muscle bundles. GPAT gene catalyzes glycerol phosphate and lipoyl-coenzyme A (CoA) to produce lysophosphatidic acid. AGPAT gene contributes to the production of diacylglycerol and DGAT gene produces triacylglycerol in the triglyceride metabolic pathway (Jarc and Petan, 2019). Differences in expression levels of these genes between animal breeds identified using RNA-seq technique have been found to be responsible for differences in intramuscular fat content and meat quality (Cheng et al., 2022; Cho et al., 2015; Cho et al., 2023; Won et al., 2023).
On the other hand, differences in intramuscular fat and meat quality could be due to feed and breed based on transcriptomic analysis. Results of transcriptomic analysis of muscles of livestock fed different feed have shown that genes are expressed differently (An et al., 2021). Biological processes affected by differentially expressed genes involve intramuscular fat and fatty acid deposition and triglyceride transport. For example, higher expression of ACSL1 gene can retard β-oxidation of fat via PPARγ signaling pathway, thereby increasing the level of triglyceride (Goh et al., 2018; Zhao et al., 2020). The FABP3 gene can specifically bind free fatty acids and facilitate esterification reactions and triglyceride resynthesis, thereby increasing the content of intramuscular fat (Xu et al., 2020). In addition, the expression of MYOD1 can inhibit adipogenic differentiation of skeletal muscle satellite cells. After feeding sheep with probiotics, MYOD1 expression is silenced, leading to increased intramuscular fat content (Zhang et al., 2022a; Zhao et al., 2022a). It has been also reported that genes related to muscle fiber compositions are differentially expressed, which can affect glycolysis and meat color (Su et al., 2019). The proportion of type I muscle fiber has been found to be high in old animals. Since type I fiber usually contains more lipids than type II fiber, meat with type I fiber is much tender (Hwang et al., 2019; Joo et al., 2013). In addition, some recent studies have reported that these differences in muscle fiber compositions can ultimately affect taste characteristics such as sourness, bitterness, and umami of meat (Hwang et al., 2020b; Komiya et al., 2020; Park et al., 2022a).
In summary, previous transcriptomic studies related to meat quality mainly focused on intramuscular fat deposition in muscles as specifically depicted in Fig. 1. Consequently, the application of transcriptomics in meat research is effective in identifying differentially expressed genes and their functions and associated signaling pathways. This can effectively describe the molecular mechanisms responsible for the altered phenotype.
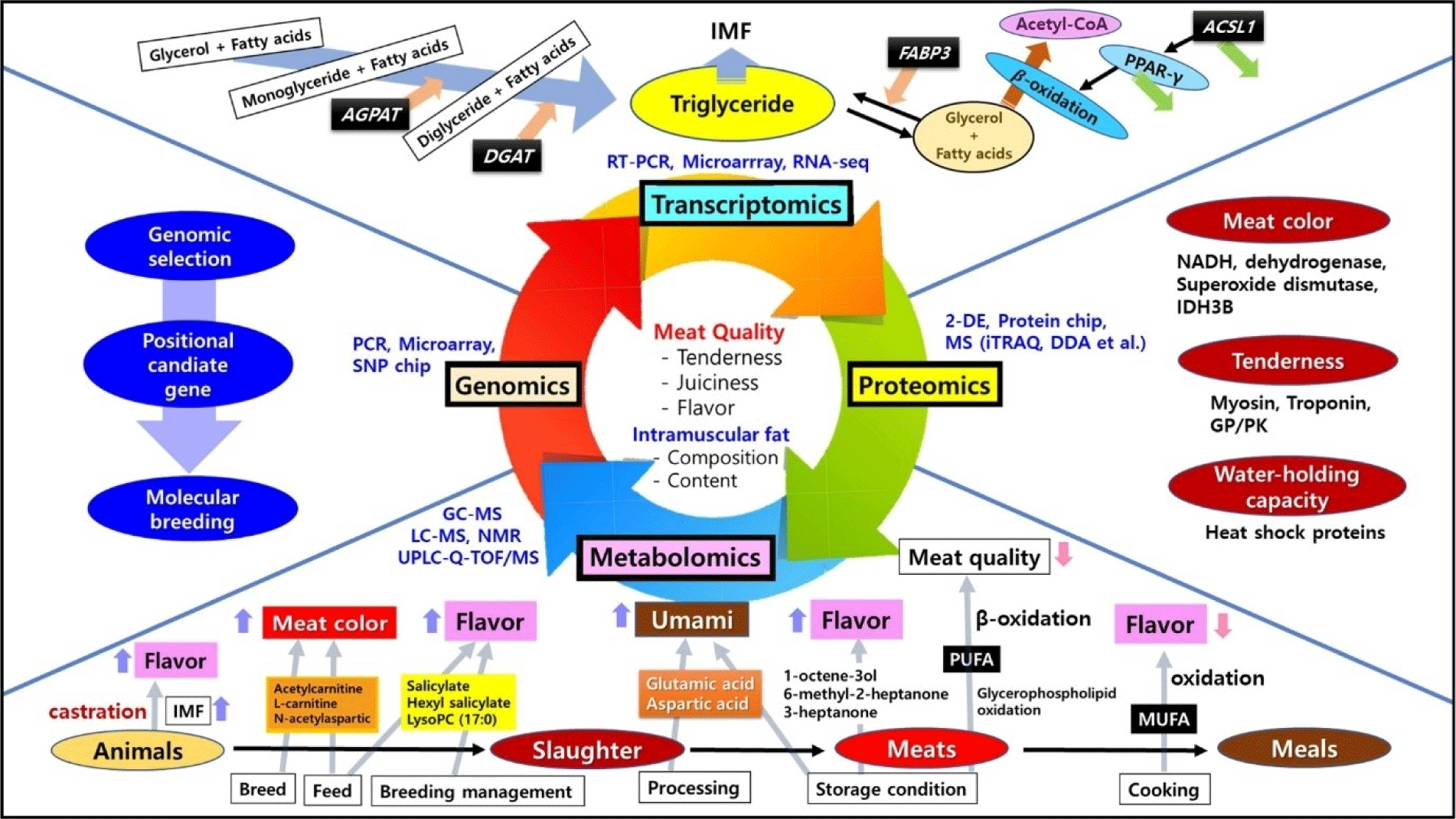
Proteomics Approach to Find Biomarkers and to Control Meat Quality
Because proteome refers to the sum of all proteins expressed in tissues or living organisms at a particular time and space, proteomics is defined as the science that characterizes the entire set of proteins expressed in cells or tissues (Aizat and Hassan, 2018; Bendixen, 2005). Proteomics uses various techniques such as electrophoresis, protein sequencing, and image statistics to visually describe the expression and function of a proteome. Proteomics tools currently used in scientific research usually include examination of protein expressions, modifications, or interactions on a large scale (Jeon et al., 2022; Kim et al., 2023c; Seo and Oh, 2023). In short, proteomics is a study that identifies and determines types and abundance of proteins, explains protein-protein interaction, and explores localization of proteins in cells and post-translational modification (Go et al., 2022; Jorrín-Novo et al., 2015; Jung et al., 2022; Kim et al., 2022b). Protein translational modifications in proteomics refers to acetylation, phosphorylation, ubiquitination, methylation, and glycosylation modification involved in protein stability, signaling that regulates protein activity, protein metabolism, and protein folding. In addition, protein modification can rapidly regulate protein activity and functions under endogenous and exogenous stimuli (Kim et al., 2022a; Kwan et al., 2016; Song et al., 2022). Since protein accounts for the largest proportion of muscle except moisture, various proteomic changes can occur during the conversion process from muscle to meat. Therefore, proteomics technology can be used to identify meat quality biomarkers.
The discovery of post-transcriptional mechanisms with direct measurement of protein expression provides useful information about biological processes and systems (Aizat and Hassan, 2018; Chevalier, 2010; Kim et al., 2023a), leading to full-scale initiation of proteomics research. Proteomics technologies have been developed through three stages: two-dimensional gel electrophoresis, protein chips, and mass spectrometry-based proteomics. Among them, current mass spectrometry is widely used in the study on proteins expressed significantly high or low in relation to protein identification, post-translational modification, and subunit-function interactions responsible for changes in meat quality. However, it is difficult to perform accurate qualitative analysis only by applying mass spectrometry. To better explain the molecular mechanisms of biochemical processes, other methods have been combined with mass spectrometry to conduct precise quantitative proteomics studies.
Over the past few years, gel-based proteomic approaches have been used to understand the molecular basis of meat quality properties. However, gel-free approaches have recently begun to gain more popularity (Ramanathan et al., 2020). The gel-free approach addresses some shortcomings associated with gel-based approaches, such as under-representation of extreme acid/basic proteins and poor sensitivity for lowly expressed proteins (Nair and Zhai, 2020). Especially, in vitro labelling techniques such as isotope-coded affinity tags (ICAT) and isobaric tags have been widely used recently. Chemically identical probes with different masses have been used to tag treatments for ICAT, with peak intensity of the first mass spectra (MS) being used to obtain the relative intensity, while the identity is derived from the second MS. Using this second MS (MS/MS), isobaric tags such as Tandem Mass Tag (TMT) and Isobaric Tag for Relative and Absolute Quantitation (iTRAQ) can obtain accurate and multiplexed quantification (Kim et al., 2023b; Zhang and Elias, 2017). In particular, TMT labeling enables multiplexing of multiple samples for relative quantification, which can improve analytical precision and accuracy.
A useful explanation of the role of protein expression changes (up-regulation and down-regulation) in the regulation of cellular activity can be provided through quantification of proteins. In other words, the search, screening, and identification of proteins related to meat quality enable accurate evaluation and rapid monitoring of meat quality. Therefore, many proteomics studies have been conducted in relation to various factors including growth environments, slaughtering methods, processing and storage methods, and protein modification in postmortem muscles that affect meat tenderness, color, and WHC. Recently, several proteomics studies have expanded our understanding of molecular and cellular mechanisms that regulate meat tenderness (Bjarnadóttir et al., 2012; Laville et al., 2009; Zhao et al., 2014), meat quality (Kim et al., 2019), meat color (Canto et al., 2015; Joseph et al., 2012; Nair et al., 2016), and WHC of meat (Kim et al., 2015).
Differentially expressed proteins (DEPs) during long-term frozen storage of meat have been investigated using iTRAQ technology. It has been found that up-regulated expression of NADH dehydrogenase and IDH3B after the first freeze-thaw cycle can promote a reduction of metmyoglobin to myoglobin and improve meat color stability (Gu et al., 2020). Enrichment analysis of DEPs has also shown that adenosine triphosphate isomerase and peroxide dismutase could be used as potential biomarkers of meat color (Aizat and Hassan, 2018). In addition, it has been found that troponin and myosin are related to meat tenderness and that heat shock proteins are water-retention proteins in meat. Moreover, it has been found that DEPs of glycolysis and ubiquitin-proteasome can cause long-term degradation of frozen meat (Kim et al., 2015). On the other hand, results of analyzing differentiated proteins in both longissimus muscles slaughtered in a non-electric shock mode and a head electric shock mode revealed that expression levels of antioxidant, binding, chaperone, and heat shock proteins were increased in sheep without electrical shock, leading to resistance to cell death (Kiran et al., 2023). It seems that DEP generated under shock and non-impact treatment can be used as an indicator of animal welfare. It has been also reported that mild slaughtering practices have a positive effect on maintaining heme integrity and high antioxidant activity, inhibit myoglobin oxidation to keep the bright meat color (Bakhsh et al., 2018; Hosseini et al., 2019). Regarding meat color changes, sarcoplasmic protein expression has been found to affect meat color stability, with more antioxidant and chaperones proteins expressed in beef color stable muscles (longissimus lumborum) than in color labile muscles (psoas major; Joseph et al., 2012). These color stable muscles show an over-abundance of myofibrillar proteins, including myosin regulatory light chain 2 and myosin light 1/3 (Canto et al., 2015). Sarcoplasmic muscle proteins such as creatine kinase M-type and triosephosphate isomerase have also been demonstrated to have positive correlations with metmyoglobin reducing activity and color stability (Nair et al., 2016).
The tenderness of meat is affected by various degrees of glycolysis in postmortem muscle cells (Warner et al., 2022). For example, glycogen phosphorylase (GP) catalyzes the degradation of glycogen to glucose-1-phosphate. The glycolysis rate is accelerated by GP activity enhanced by the phosphorylated form (Li et al., 2017). In the glycolytic pathway, pyruvate kinase (PK) acts as a rate-limiting enzyme and catalyzes phosphoenolpyruvate with pyruvate and adenosine triphosphate (ATP). Because phosphorylated PK is highly active, high levels of phosphorylation can promote glycolysis, resulting in tougher meat (Li et al., 2021). In addition, phosphorylated proteins are involved in the degradation of calpain proteins and Z-disc-related proteins, the stability of myoglobin, and the dissociation of actomyosin by regulating the glycolysis rate (Chen et al., 2018; Huang et al., 2020). On the other hand, as thermal processing induces protein oxidation which affects meat texture, DEPs could be identified according to heat treatment. DEPs can include structural and metabolic proteins such as myosin known to undergo oxidative deformation when heated, resulting in tougher meat. In addition, during the cooking process of meat, phosphorylation of PK is promoted and glycolysis is accelerated, causing greater oxidative stress in muscles and making the meat tougher. A decrease in troponin T and an increase in the glycolytic enzyme during boiling treatment can promote tenderness of meat (Jia et al., 2022).
In summary, proteomics has been widely applied to meat quality research and finding relevant biomarkers (Fig. 1). A comprehensive interpretation of results of meat quality studies through various proteomic techniques will reveal methods of breeding meat animals that can produce better meat quality (Gagaoua et al., 2022).
Metabolomics Approach to Improve Meat Quality and Taste Characteristics
Metabolomics is defined as systematic analysis of small molecules such as amino acids, fatty acids, and glycolytic or tricarboxylic intermediates produced in the biological system (Fiehn and Weckwerth, 2003; Ramanathan et al., 2020). A metabolome is a complete set of metabolites of biological sample with a low molecular weight of less than 1,000 Da. Metabolomics explores metabolic mechanisms by qualitatively and quantitatively analyzing metabolites affected by internal and external environments using high-throughput techniques (Baharum and Azizan, 2018). These metabolomic analyses involve the study of metabolites, the entirety of endogenous small molecules within an organism, organ, biological tissue, or cells (Fiehn and Weckwerth, 2003; Vasquez et al., 2022). Measuring real-time changes in metabolites is helpful to understand ultimate phenotypical changes caused by changes in environment or gene expression in biological systems (Huang et al., 2022; Muhizi et al., 2022; Park et al., 2022b). Thus, during meat production, an understanding of the direct correlation between metabolites and biological phenotypes that vary according to breed, feeding, and storage conditions can be effectively used to improve meat quality and taste characteristics.
Metabolomic analysis requires a high level of techniques because chemical properties metabolites vary according to biological environment conditions (Straadt et al., 2014; Zhang et al., 2012). Since metabolomics analysis quantifies only about 15%–30% of total metabolites present in a system (Misra et al., 2018), metabolomic analysis using a single platform is bound to limitation as it might only generate limited information (Gagaoua et al., 2021). Common analytical tools used to separate various metabolites include gas chromatography, liquid chromatography, capillary electrophoresis, and nuclear magnetic resonance (NMR; Baharum and Azizan, 2018; Eom et al., 2022; Harlina et al., 2022; Kwon et al., 2022; Ramanathan et al., 2020; Wang et al., 2022).
Metabolomic analysis involves the usage of either a single platform or a combination of platforms to separate molecules. Popular analytical tools to separate various metabolites include NMR, gas chromatography-mass spectrometry (GC-MS), and liquid chromatography-mass spectrometry (LC-MS; Baharum and Azizan, 2018; Ramanathan et al., 2020). The combination of mass spectrometry with gas chromatography and liquid chromatography is routinely used in meat science research as the use of mass spectrometry helps identify and characterize isolated molecules (Ramanathan et al., 2020). In general, GC-MS is used to measure volatile compounds such as aldehyde, ketone, and alcohol, while LC-MS is used to detect amino acids, fatty acids, and other non-volatile compounds. NMR is also used to understand nutrient states associated with meat quality by measuring molecular relaxation time and to distinguish meat quality. With recent development of metabolomic technology, the process of identifying metabolites has developed more profoundly. Samples can be analyzed by selecting an appropriate method among NMR, GC-MS, and LC-MS according to the required detection results. Currently, metabolomics techniques are being applied to study effects of pre-slaughter factors (such as breed, feed and breeding management) and postmortem factors (such as processing and storage conditions) on meat quality.
Metabolites such as lipids, fatty acids, and volatile compounds are expressed differently depending on the breed of livestock. More expression of amyl salicylate, hexyl salicylate, and LysoPC (17:0) can increase the pleasant flavor of meat. Expression of alkaloids such as (E)-scalamide, murrayanine, and nigakinone increases the bitter taste intensity of meat, while the expression of deoxyiso-cucurbitin inhibits the bitter taste. The expression of arachidonic acid gives a stimulating and sweet taste (Wang et al., 2019b). However, if polyunsaturated fatty acids (PUFA) and monounsaturated fatty acids are oxidized during cooking, flavor defects may occur (Kosowska et al., 2017). On the other hand, since most alkaloids expressed in meat are derived from diet, meat quality and palatability can be improved by controlling dietary compositions of livestock. Meat color can be improved through dietary control because the expression of acetylcarnitine, L-carnitine, and N-acetylaspartic acid, which can regulate the synthesis of myoglobin according to diets, is affected (Wang et al., 2021a). Depending on the diet, the expression of carnosine and creatinine can affect the bitterness and sourness of meat, respectively. In particular, the expression of sweet and umami amino acids such as glutamic acid and aspartic acid is affected by the diet (Hwang et al., 2020a; Jeong et al., 2020). In addition, studies are being conducted to explore biomarkers that can represent meat freshness because changes in metabolites that occur during refrigerated storage of meat have a significant impact on meat quality and palatability (You et al., 2018). As the refrigeration period increases, the degradation of glycogen and proteins increases the synthesis of alanine, aspartic acid, and glutamic acid. Tricarboxylic acid cycle (TCA) cycle, amino sugar biosynthesis, and nucleotide metabolic pathways are also activated to produce 1,5-dehydrated glucitol (Wu et al., 2023). These can cause decrease of pH of meat, activating endogenous proteases and accelerating meat spoilage. Since these metabolic studies provide meaningful data for characterization and freshness prediction of meat, metabolic techniques not only can suggest ways to improve meat quality, but also can help explain effects of breed, feed, breeding management, slaughter, processing, and storage on meat metabolites (Muroya, 2023).
Metabolomic analysis related to flavor and taste characteristics of meat is mainly conducted on metabolites of lipids and proteins that can be divided into lipidomic and flavor metabolomics (Aung et al., 2023). Lipidomics studies cellular lipid metabolism by investigating lipid components and contents, while flavor metabolomics intensively studies metabolic processes of volatile compounds and amino acids (Zhang et al., 2022b). Lipid-rich meat is sensitive to oxidation. It provides a distinctive flavor of each livestock species or breeds during production and storage, which is affected by breed, nutritional ratios in feed, breeding management, slaughter, processing, and storage conditions. High-energy/high-fat feed can promote the synthesis of lipids in livestock muscles and increase levels of L-carnitine and acetyl-carnitine, while lowering contents of inosine monophosphate and taurine can decrease meat color stability (Wang et al., 2021b). Expression levels of lipid-derived compounds such as 1-octen-3-ol, 2-pentyl furan, nonanal, heptanal, and butanal are highly correlated with low intramuscular fat (IMF) content, while the expression of dimethyl trisulfide is correlated with a high IMF content (Li et al., 2022). On the other hand, castration of animal can improve the flavor of meat by increasing IMF content, and decreasing the level of ketone with increasing the level of alcohol, aldehyde and ester (Li et al., 2020).
The expression of metabolites also varies depending on meat storage method. During early stages of refrigerated storage at 4°C, levels of 1-octene-3-ol, 6-methyl-2-heptanone, and 3-heptanone are increased, causing meat to have a floral, fruity, or creamy flavor (Xu et al., 2023). At this time, changes in acylcarnitine are related to increases of in unsaturated fatty acids, which means that the difference in flavor of meat before and after storage is related to beta-oxidation of unsaturated fatty acids. In particular, unsaturated fatty acids in triglycerides are important substances that can affect meat flavor during early stages of storage while oxidation of triglycerides plays a role in late stages of storage. Lipid metabolites that are expressed differentially during meat storage at 4°C are mainly due to oxidation of glycerophospholipids and fatty acyls, which eventually increases contents of PUFA (Zhang et al., 2023). In addition, oxidation of PUFA produces volatile carbonyl and accumulation of PUFA promotes mitochondrial uncoupling, continuously increasing the content of acylcarnitine, which undergoes β-oxidation and causes cellular heat production, thus reducing meat quality. In addition, storing meat at –20°C for 12 days increases the ratio of long-chain acylcarnitines to L-carnitine. It also increases fatty acid oxidation. For the next 12 days, β-oxidation of fatty acids decreases, the conversion of phospholipids to sphingolipids occurs, the self-oxidation rate of meat reaches food quality limit, and the bitterness intensity of meat increases throughout the storage period (Jia et al., 2021a). Various lipid metabolites are measured according to the cooking method of meat. Boiling treatment can promote the degradation of phosphatidylcholine (PC) into sphingomyelin and steaming treatment can reduce the loss of PC and lysophosphatidylcholine (Jia et al., 2021b). Regarding the effect of baking on lipid profiles, PC (30:6) and PC (28:3) are decreased rapidly by 5 minutes of roasting, increased significantly from 5 minutes to 7.5 minutes, and then decreased again from 7.5 minutes to 15 minutes to show its potential as a molecular biomarker for roasting meat (Liu et al., 2022).
Fig. 1. shows a brief schematic diagram of metabolic markers and pathways related to meat quality. Research in lipidomics and flavor metabolomics can sufficiently identify biomarkers that control meat quality. Analysis of meat quality and various biomarker lipids can enhance understanding of major processes and mechanisms affecting meat lipid and flavor, including biosynthesis of unsaturated fatty acids, lipid oxidation, and lipid denaturation. In other words, biomarker lipids can be a reference to characterize lipids of meat, which allows us to better understand meat quality.
Multi-Omics Approach to Improve Meat Quality and Taste Characteristics
As shown in Fig. 2, multi-omics approaches use a combination of individual omics data tools in a sequential or simultaneous manner to bridge the genotype-to-phenotype gap (Kuo et al., 2013). The reason why multi-omics can better explain biochemical changes in meat than a single omics is that all biomolecules such as DNA, RNA, proteins, and metabolite functions are interrelated. Genes that determine the ability to produce high-quality meat appear phenotypes through transcription, translation, post-translational modification, and metabolism. Therefore, molecular mechanisms responsible for changes in meat quality can be explored and decoded with the aid of multi-omics that integrate genomics, transcriptomics, proteomics, and metabolomics (Ramanathan et al., 2020).
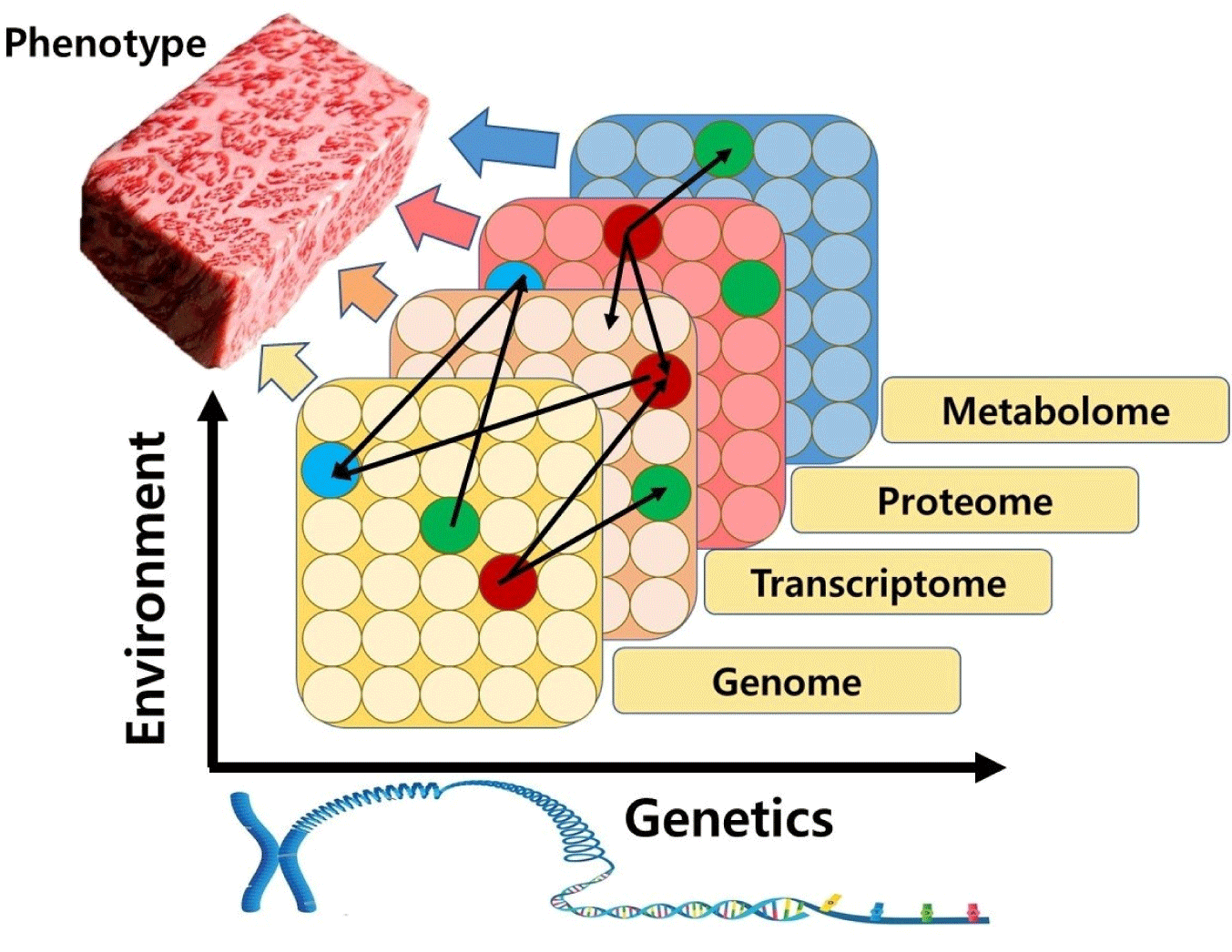
Multi-omics is based on bioinformatics analysis to identify expression differences in big data and to predict potential biomarkers (Kim and Kim, 2021). Multi-omics analysis enables prediction of biological functions by associating omics data with biological phenotypes through cluster analysis. In addition, interactions between RNA/protein/metabolite can be predicted by correlating omics data with related pathway mechanisms (Jung et al., 2020). Thus, despite the potential of single omics, multi-omics can deepen our understanding of postmortem metabolism, thereby developing better strategies to help develop potential meat quality biomarkers.
A major issue in integrating all omics is big data processing, which allows some software to be used. However, it has limitations in data integration. In recent years, the integration of different omics technologies to explain changes in food quality on a molecular basis has been implemented in various research areas such as systems microbiology (Fondi and Liò, 2015), food and nutritional science (Kato et al., 2011), disease biology (Pathak and Davé, 2014), and meat science (Lim et al., 2017; Ma et al., 2020; Windarsih et al., 2022; Yan et al., 2021). Although successful integration has not yet been realized in meat science research, studies have been conducted by integrating transcriptomics and proteomics (Lim et al., 2017; Yan et al., 2021; Zhang et al., 2022c; Zhao et al., 2022b), proteomics and metabolomics (Ma et al., 2020; Windarsih et al., 2022), and transcriptomics and metabolomics (Chen et al., 2022; Cui et al., 2023; Wu et al., 2020; Zhang et al., 2022d).
In general, most tools utilized in proteomics are extended to genomics data. Genomics data are usually analyzed using DNA microarray technology (also called gene expression profiling; Berger et al., 2013). Correlation between mRNA and protein is analyzed using changes in mRNA and protein expression levels. Pathway analysis of genes having a consistent, opposite, or unrelated expression tendency is performed to obtain an analysis of expression patterns of mRNAs and proteins. Pathway and interaction network analysis of differentially expressed genes and proteins is based on molecular functions, subcellular localization, and biological processes (Salovska et al., 2020). This combination analysis of transcriptome and proteome is mainly focused on research related to fat deposition in muscles. Differential genes and proteins involved in lipid deposition and amino acid synthesis in meat have been identified and understanding of the tenderizing mechanism and flavor of meat has increased (Lim et al., 2017).
The main purpose of an integrated analysis of proteome and metabolites is to find regulatory association between proteins and metabolites. A representative integrated study of proteomes and metabolites related to meat quality research has been conducted using callipyge lambs. It was found that differentially abundant proteins and metabolites were mainly energy metabolism enzymes and coenzymes, apoptosis-related factors, and antioxidant metabolites compounds (Ma et al., 2020). Specifically, decreased abundance of pro-apoptotic proteins such as cytochrome C and overexpression of anti-apoptotic proteins such as HSP70, BAG3, and PARK7 can cause delayed apoptosis, making callipyge lamb tough. In addition, it was confirmed from enzymes expressed high or low in glycolysis and TCA cycle that callipyge genes promoted the shift of muscle to higher fast contraction and glycolytic muscle fiber composition. On the other hand, meat research studies that combine metabolomics and transcriptomics have mainly focused on how fat deposition is regulated and improved in tenderness by multiple genes and signaling pathways. High-fiber diets can inactivate genes associated with steroid hormone-mediated signaling pathways such as NR1D1, NR4A1, and NR4A2 through epigenetic modification. Then retinoic acid regulates glucose metabolism and induces gluconeogenesis. When blood sugar content decreases, fat content in muscles decreases. In metabolomics related to glucose metabolism, it has been found that phosphoenolpyruvate and glucose 6-phosphate are involved in gluconeogenesis and that methylbutyric acid can reduce the rate of fat deposition. Studies that combine transcriptomics and metabolomics have revealed that although a high-fiber diet can promote muscle fiber growth to a certain extent, it can lead to meat toughness (Wu et al., 2020). Recently, multi-omics of transcriptomics and metabolomics have been increasingly used to study effects of various nutritional factors on meat quality.
Summary and Future Perspectives
Omics analysis is very beneficial for exploring mechanisms and underlying pathways associated with meat quality and safety, nutritional properties of protein and fat, and health impacts. Genomics and transcriptomics enable the screening of differentially expressed genes for molecular breeding to obtain high-quality and delicious meat. Proteomics provides a comprehensive understanding of the molecular mechanisms that affect meat quality, enabling accurate control of meat quality. Metabolomics enables the analysis of small metabolites involved in real-time changes in meat quality affected by various factors. Consequently, integrated multi-omics can explain differences in meat quality more collectively through mutual verification of results from individual omics.
Since multi-omics depend on high-quality databases, meat quality assessment and prediction models for discovered biomarkers related to meat taste and safety should be established. In addition, optimization and expansion of existing databases can provide baselines that increase profitability of the meat industry and produce high-quality meat and meat products for consumers. However, to summarize recent multi-omics studies related to meat quality, some challenges need to be addressed. First, although stable nuclide tracing techniques can efficiently identify meat quality control-related mechanism because levels of changes in intracellular substances can be well known, these techniques are not currently used. Not only does searching for biomarkers using multi-omics generally make it difficult to find them for single meat quality, but also multi-omics is a robust tool for high throughput screening, making reasonable validation difficult when it is used for data annotation and enrichment. Furthermore, while the accuracy and preference of multi-omics technologies affect authenticity to a certain extent, current database of multi-omics lacks complete data. In addition, efficient and scientific methods for correlation and integrated analysis of datasets obtained from multidimensional omics studies are still lacking.
To address these challenges of multi-omics, the application of tracer techniques to meat quality research must be strengthened and meat quality regulatory mechanism must be better explained by focusing on dynamic changes of intracellular substances. When using multi-omics techniques to explore meat quality-related mechanisms, potential molecular biomarkers should be validated in a reasonable manner and state-of-art omics technologies such as third-generation transcriptome sequencing, “4D” proteomics, and spatial omics should be applied to future meat quality studies. In addition, a multi-omics database with genes as connection points should be established. Integrated algorithm and software for multi-omics data should also be developed.