Introduction
Recently, per capita income has improved in Asian countries such as Japan, Korea, and China. Consumption of processed meat products has also increased along with an increase in consumption of fresh meat. In particular, consumption of meat has been significantly increased due to the rise of per capita gross national income (GNI). Pork is an important food with the largest share in the consumption of meat (Kim et al., 2017). Per capita consumption of pork in Korea has increased from 16.5 kg in 2000 to 22.5 kg in 2015 (Korean Statistical Information Service, KOSIS). Annual production of processed meat products has increased significantly from 110,290 tons to 182,462 tons in the same period. Production of domestic pork (PDP) and import of pork (IP) has greatly increased as a result accompanied by increased consumption of pork and meat products. After free trade agreements (FTA) between Korea and Chile in April 2004, as Korea concluded FTA with many countries such as EU and US (Korea Meat Trade Association, KMTA), import of processed meat products has increased more than twice from 6,080 tons in 2004 to 13,457 tons in 2015 in Korea.
The primary goal of meat industry is to produce high quality meat from livestock of various species. However, the meat itself can not satisfy various consumer needs (Jin, 2017). Therefore, meat products have been developed to satisfy various consumer needs and improve storage of fresh meat. Meat product has been mainly developed in Europe such as Germany. Meat products are positioned as important foods for people in America such as the United States and Canada. Raw materials used for processed meat products include beef and pork, for which 1.1% and 6.5% are produced in Korea (KMTA), respectively. Therefore, domestic-produced pork is a more important factor than domestic-produced beef for manufacturing processed meat products (Jin, 2017). In Korea, pork consumption is in short supply owing to excessive preference of pork belly and foreleg. Accumulation of total stock in non-favorable areas such as ham and loin causes management deterioration for producers and distributors. Therefore, non-favorable cuts need to be explored for utilization through analysis of correlation between production volume of various meat products and supply quantity of domestic pork. In particular, in terms of the 6th industrialization of agriculture and livestock industry that is consistently carried out in production, processing and distribution or sales is currently performed in Asia to improve competitiveness. Logical analysis of the relationship between domestic supply of pork and processed meat products produced in Korea plays a role as an important indicator for decision making of entrepreneurs to promote the 6th industrialization of pig industry.
Canonical correlation analysis (CCA) is a useful statistical method for analysis of relationship between sets of variables containing two or more variables. CCA derives an equation to combine linear variables from correlation of variables in a respective set and then analyzes their correlation from the derived equation (Kim et al., 2017). CCA is the most appropriate method when a researcher desires to examine the relationship between two variable sets (Sherry and Henson, 2005). CCA has been used in various fields, including psychology, social science, political science, ecology, education, sociology, physical sciences, tourism, and marketing (Kim et al., 2017).
Kim et al. (2017) introduced CCA to predict relationship between color and physicochemical traits in pork. The canonical correlation coefficient was 0.819, showing significant relationship between the first pair of canonical variates (p<0.01). To produce reddish pink color in pork preferred by consumers depending on relationships between pork quality traits, they suggested that producer should have maintain proper post-mortem pH 24 h, higher WHC, lower drip loss, and lower cooking loss for pork.
Ventura et al. (2011) have applied CCA to examine the association between carcass and ham traits in a pig population used to produce dry-cured ham. Canonical correlations between carcass and ham traits at 160 kg were 0.77, 0.24, and 0.20 for the first, second, and third canonical pair, respectively, all of which showed significant values (p<0.01). They have suggested that cull pig is not generally suitable for dry-cured ham production. However, it is possible to apply it to meat products.
Cankaya et al. (2008) have applied CCA to estimate the relationship between three different sexual maturity traits and level of nutrient intake or egg production traits at two different periods. If contribution of differences in nutrient intakes were eliminated from onset of egg production, they suggested that the effect of body weight at sexual maturity upon availability of nutrients had a higher contribution to variation of egg production in pullets.
Mendes and Akkartal (2007) have investigated relationships between pre- and post-slaughter traits of Ross 308 male chickens. Results of their correlation analyses were significant only in the first canonical correlation for both ages (p=0.035 and p=0.0013). Correlations showed higher value in the fourth week of age than those in the second week of age. Their results suggested that live body weight, shank length, and breast width were clearly positively correlated with hot carcass weight, beck weight, and right rump weight at both ages.
Cankaya and Kayaalp (2007) have applied CCA to estimate the relationship between eight different morphologic characters and live weights at three different periods. The estimated canonical correlation coefficient (0.931) showed significant value between the first pair of canonical variables (p<0.01). They suggested that measured chest depth and chest girth at weaning period could be used as early selection criteria for increasing live weights of German Fawn Hair Crossbred goats.
Akbas and Takma (2005) has applied CCA to estimate relationships of egg production with age at sexual maturity, body weight, and egg weight. CCA was designed to evaluate the relationship between two sets of variables of laying hens. Estimated canonical correlations showed significant relationships between the first and the second pair of canonical variates (p<0.01). Canonical weights and loadings from CCA indicated that age at sexual maturity had larger contribution than body weight and egg weight to variation of the number of egg productions at three different periods.
The purpose of this study was to estimate associations between variable sets related to supply quantity of pork and the production of processed meat products in Korea by CCA. Results of this study will be useful for making policy decisions to utilize ham and loin classified as non-favorable primal cuts of pork in Korea.
Materials and Methods
Secondary data applied in this study were collected from Korea Meat Trade Association (KMTA) and Korea Meat Industries Association (KMIA). All data were monthly classified from January 2003 to December 2016 (168 months). PDP, beginning stock of domestic pork (BSDP), and IP among collected data were selected as dependent or criteria variable set owing to characteristic of supply function for pork. Otherwise, production of ham (PH), production of sausage (PS), production of bacon (PB), production of can ham (PC), and production of mix sausage (PMS) were selected as independent or predictor variable set owing to production of processed meat products.
CCA is a suitable technique that can establish interrelation between two sets of variables as well as quantify the percentage of variance common to the two sets (Kim et al., 2017; Ventural et al., 2011). CCA indicates correlation between two linear combinations of sets of dependent and independent variables as linear combinations of variables useful for predictive or comparative purposes (Akbas and Takma, 2005; Sahin et al., 2011). Therefore, the goal of CCA is to find the best linear combination between two multivariate datasets that can maximize the correlation coefficient between them (Malacarne, 2014). Linear combinations of original variables can be defined by canonical variates (Vi and Wi) as follows:
The correlation between Vi and Wi can be defined to canonical correlation. CCA is repeatedly looking coefficients a and b to maximize the correlation between Vi and Wi. The maximum number of canonical function that can be extracted equals to the number of variable in the smallest canonical variate (Dattalo, 2014) which is three in this study (a number of pork supply quantity variables). The first canonical function is derived to maximize the correlation between Vi and Wi variables (Laessig and Duckett, 1979).
Standardized canonical coefficients and canonical loadings were used to evaluate the relative importance of variables in the model (Dattalo, 2014). Standardized coefficients are interpreted similar to standardized regression coefficients in multiple regressions. Therefore, CCA is used to estimate canonical coefficients (ai1, ai2, …, aip and bi1, bi2, …, biq) when the canonical correlation is at the maximum (Akbas and Takma, 2005). Canonical loading reflects the variance that the observed variable can be shared with canonical variate and interpreted like a factor loading in assessing the relative contribution of each variable to each canonical function (Safari et al., 2013). Redundancy index (RI) is proposed to calculate each canonical correlation to determine how much of the variance in one set of variables is accounted by the other set of variables (Kim et al., 2017; Safari et al., 2013; Sharma, 1996).
Results
In domestic pork supply variables set of pork, PDP decreased temporarily due to the outbreak of foot-and-mouth disease that occurred at the end of 2010, but it has increased since then. Pork imports and carryover volumes have been increasing steadily over time due to the effects of the FTA (Fig. 1). In particular, it is examined that the IP rapidly increased temporarily when production of domestic pork decreased sharply due to domestic foot and mouth disease.
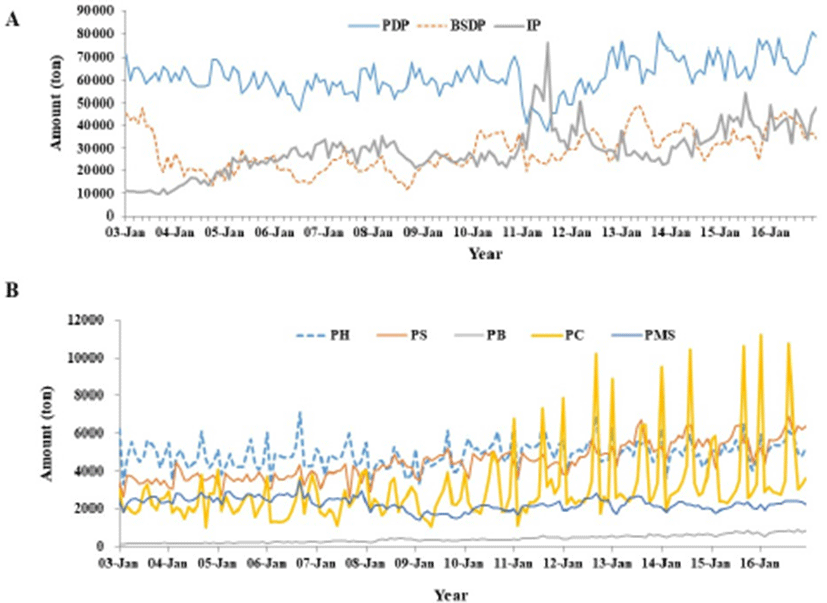
In the set of processed meat products, PS, PB, and PMS remain stable without major fluctuations except for seasonal fluctuations, whereas PC shows a trend of increasing with seasonal variation. PS has shown a slight increase trend continuously, which contributes greatly to the growth of domestic meat products.
The collected data were presented by descriptive statistics for factors indicating supply quantity of pork and the production of processed meat products (Table 1). The volume of domestically distributed pork was 61,773, 28,772, and 29,037 tons from PDP, BSDP, and IP, respectively, with a total volume of 119,582 tons. The volume of PDP accounted for 51.7% of total pork production, which was 2.2 and 2.1 folds higher, respectively, than those of BSDP and IP. Supplies of BSDP and IP were maintained at a similar level.
Otherwise, volumes of processed meat products were 4,927, 4,512, 403, 3,061, and 2,275 tons from PH, PS, PB, PC and PMS, respectively, with a total volume of 15,178 tons. The production of PH had the highest amount whereas that of PB had the lowest volume. Processed meat products accounted for 12.7% in volume of total pork production. Therefore, many parts of domestically distributed pork were used not for processed meat, but for fresh meat itself.
Shares of production and sale volumes of majorly processed meat products are shown in Fig. 2. Productions of sausage and ham accounted for 34.3% and 28.5% of domestic production, respectively, which occupied 63.3% of total production in June 2016. Otherwise, they accounted for 36.8% and 29.2% of domestic sales, respectively, which occupied 66% of total sales (KMIA). In addition, the production of meat products in Korea was dominated by oligopoly, accounting for 75.6% of total production by the top five companies of 110 manufacturers in 2012 (KATC, 2013). Majorly processed meat products were mainly sold on large marts and chain super-markets. However, the market share of special department maintained at a very low level (KATC, 2013).
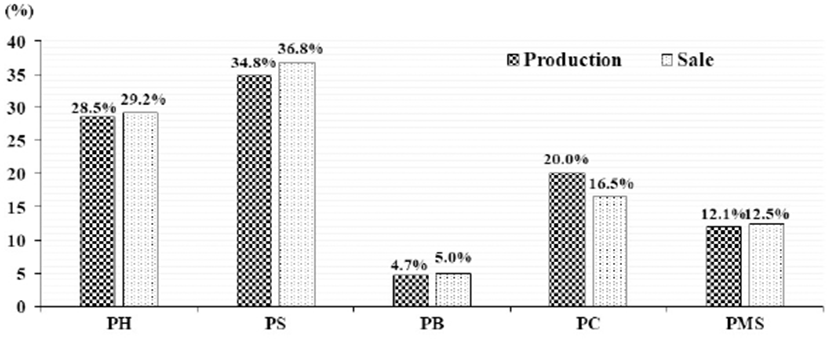
Bivariate correlations among supply variables of pork and production variables of processed meat products in Korea are shown in Table 2. Results showed correlations between variable sets of PDP, BSDP, or IP (determining the amount to be supplied to the domestic pork market) and variable sets of PH, PS, PB, PC or PMS (determining domestically produced and processed meat products). Among relevant variables for pork supply, PDP had a significant correlation with production variables of domestically processed meat products such as PS and PB. In addition, BSDP and IP showed significant relationships with PH, PS, PB, and PC. Especially, IP showed high correlations with production variables of domestically processed meat products (p<0.01). Conversely, PMS maintained a few correlations with supply variables of domestic pork.
However, it is impossible to simultaneously explain the relationship between the set of variables that determined domestic pork supply and the set of variables that determined the production of domestic meat product. Therefore, in this study, CCA was introduced to explain the relationship between supply variables of pork and production variables of processed meat products in Korea.
Results from CCA for three pairs of canonical variate due to variables of three supply factors for pork in the smaller variable set are shown in Table 3. The first canonical correlation was 0.8576. It represented the highest possible correlation between any linear combinations for supply factor variables of pork (V1) and production factor variables of processed meat products (W1) in the market (p<0.01). The second canonical correlation was 0.3497. It indicated that the relationship between canonical variates was significant (p<0.01). However, canonical correlations only measured correlations between linear combinations of variables rather than directly measuring correlations between two sets of variables (Kim et al., 2017). Therefore, canonical correlations tend to be high between two original sets of variables that are sometimes exaggerated (Laessig and Duckett, 1979).
Standardized canonical coefficients for pairs of all canonical variables (V1 and W1) are shown in Table 4. Canonical variates representing optimal linear combinations of dependent and independent variables are defined by standardized canonical coefficients as follows:
V1, V2 and V3, 1st, 2nd and 3rd supply variables; W1, W2, and W3, 1st, 2nd and 3rd processed meat production variables; PDP, production of domestic pork; BSDP, beginning stocks of domestic pork; IP, imports of pork; PH, production of ham; PS, production of sausage; PB, production of bacon; PC, production of can ham; PMS, production of mix sausage.
Standardized canonical coefficients of IP, PDP, and BSDP as supply variables of pork were 0.7578, 0.4136, and 0.3237, respectively, suggesting that they contributed importantly to the first canonical variate (V1). On the other hand, the coefficient of PB as production variable of processed meat products was 0.9382, indicating that it contributed the largest to the first canonical variate (W1).
Canonical loadings of original variables with their canonical variables are shown in Table 5. Canonical loading presents product-moment correlation between the original variable and its corresponding canonical variate (Kim et al., 2017). These values reflect the degree of a variable to be represented by a canonical variate (Tahtali et al., 2012; Yaprak et al., 2008). Canonical loadings for supply variables of pork suggested that IP (0.7864) and BSDP (0.6182) had more effect than PDP (0.4931) to form the first fair for supply quantity variables of pork (V1). On the other hand, canonical loadings for PB (0.9988) and PS (0.8945) had stronger effects compared to other factors to form the first fair for production variables of processed meat products (W1).
V1, V2 and V3, 1st, 2nd and 3rd supply variables; W1, W2, and W3, 1st, 2nd and 3rd processed meat production variables; PDP, production of domestic pork; BSDP, beginning stocks of domestic pork; IP, imports of pork; PH, production of ham; PS, production of sausage; PB, production of bacon; PC, production of can ham; PMS, production of mix sausage.
Related structure of the first canonical correlation on V1 and W1 is illustrated in Fig. 3 (also see Tables 3 and 5). Numbers of dimension explaining the relationships between variable sets were reduced from 8 to 2 by CCA. These results indicated high correlation between set of pork supply variables (V1) and set of the production variables of processed meat products (W1) (at 85.76%). Cross loadings represent correlations between original variables and opposite canonical variables (Kim et al., 2017). According to cross loading results, the first pair of canonical variables IP (0.6744) and BSDP (0.5302) provided relatively strong contribution to canonical variate W1 whereas PB (0.8566) and PS (0.7671) highly contributed to V1 (Table 6).
W1, W2, and W3, 1st, 2nd and 3rd processed meat production variables; V1, V2 and V3, 1st, 2nd and 3rd supply variables; PDP, production of domestic pork; BSDP, beginning stocks of domestic pork; IP, imports of pork; PH, production of ham; PS, production of sausage; PB, production of bacon; PC, production of can ham; PMS, production of mix sausage.
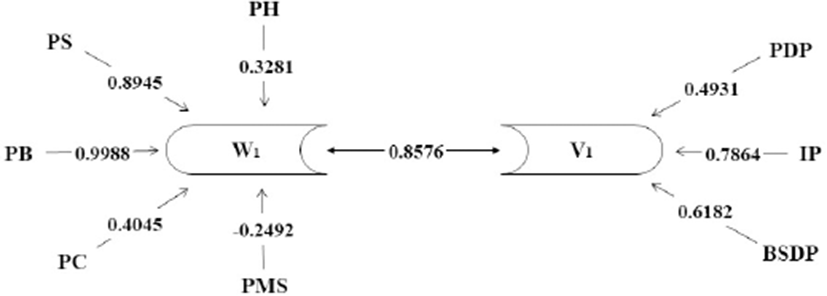
Therefore, IP and BSDP not only gave much stronger influence on the related structure of canonical correlation on X variable set (Vi) than PDP, but also provide strong contribution to the related structure of canonical correlation on Y variable set (Wi). On the other hand, PS and PB led to a change in the production volume in domestic meat product. They were matched with a changing trend of domestic supply of pork compared to the production of other processed meat products.
Canonical redundancy analysis examines how original variables can be well-predicted from canonical variables (SAS, 2009). RI indicates total proportion of variance to be shared by two sets of original variables. It was designed to overcome inflated correlations (Laessig and Duckett, 1979). Results of analysis for canonical redundancy in this study showed that 41.46% of shared variance in supply quantity factors (X variable set) was explained by the first pair of canonical variate V1 (Table 7). Otherwise, RI of 0.3049 for the first canonical variable was explained by 30.49% of the proportion for canonical variate W1 where RI (0.3049) was derived by multiplying shared covariance (0.4146) and squared canonical correlation (0.7356). In addition, 31.35% of total variation in production factors of processed meat products (Y variable set) was explained by the first pair of canonical variate W1. RI of 0.3135 for the first canonical variate was explained by 31.35% of the proportion for canonical variate V1. Thus, canonical redundancy analysis explained 30.49% and 31.35% of variance, suggesting that the pair of canonical variables can overall well predict the opposite set of variables.
Discussion
Recently, improvement in expendable income of consumers has led to a trend to stimulate more demand not only for quality, but also for quantity of meat products (Adesehinwa et al., 2003). Consumer’s purchasing power is known to be an important factor that can induce activation of modern pork industry (Suckling, 2012). In other words, when disposable income of consumer is increased, consumption of fresh pork and processed meat products is also increased (Kim et al., 2017). Sustainable livestock production to provide livelihood and ensure food or nutrition to a large population is dependent on efficient utilization of livestock products. Value-added meat product production contributes to sustainable demand and efficient marketing of meat with reasonable income from meat animals for farmers (Kondaiah, 2004).
Countries with high consumption of meat such as the United States prefer comparatively low-fat cuts of pork with high nutrition. However, Korean consumers who are accustomed to roasted meat prefer relatively high-fat cuts such as pork belly and shoulder butt (Choi and Cho, 2011). Inventory levels of low-fat cuts are accumulated owing to the consumption pattern in Korea, resulting in inequality between supply and demand of pork with increase of social costs. Therefore, expanding market for meat products to apply low-fat cuts has a very important meaning in socio-economic aspect. In addition, consumers are increasingly demanding ready-to-eat and ready-to-cook meat products with better quality and taste. Customer’s awareness and acceptance regarding the convenience of meat product is an important factor in the growth of processed meat industry (Fernando, 2017). Since the processing industry plays an important part in the value chain and largely determines the competitiveness of agricultural industry, agricultural policy should aid the processing industry (Rudnskaya, 2017).
According to the results in this study, a canonical correlation of 85.76% was shown between variable sets of domestic pork supply and meat products (Table 3 and Fig. 2). In addition, PDP, BSDP, and IP for determining domestic pork supply amount showed significant correlations with PH, PS, PB, and PC for determining domestic meat processed products (Table 2). Especially, the production of processed meat products had a high relationship with IP and BSDP (Tables 3, 5, 6, and Fig. 2). These results indicate that domestic pork produced in the current month is more likely to be traded for fresh meat than to be manufactured for processed meat products. This suggests that inexpensively imported pork has a high probability to be manufactured as meat products to be supplied into the market. Although Korean consumers require processed meat products based on domestically produced pork (Choi and Cho, 2011), meat processors apply the imported raw pork for processed meat products because of the high price and unstable supply of domestic raw pork. In addition, imported raw pork showed relatively higher handling share than beef and chicken.
Therefore, it is necessary to lower the price of domestically produced pork for application as a raw material to expand consumption of domestic meat products. However, this is practically difficult because it can lead to economically damage to pig farmers. Accordingly, a system that can stably supply raw pork needs to be constructed for processed meat products. For this purpose, it is necessary for meat processors to directly produce raw meat or strengthen contract transactions.
In addition, various products and distribution channels are required to satisfy needs of various consumers while weakening oligopoly of market for domestic meat products. Recently, consumers are seeking health-oriented consumption while the aging population and nuclear family are accelerating. Therefore, it is necessary to produce and supply processed meat products with quality, hygiene, and functionality required by diversified consumers.
Finally, related regimes need to be improved in order to revitalize the market of processed meat products. For processed meat products such as handmade ham and large sausage, Europe, America, and Japan have promoted consumption to emphasize product diversity and image of fresh meat products by permitting sales to be divided within the sale hall (Choi and Cho, 2011). Korea has improved the regime so that meat products such as ham and sausage are sold with fresh meat at a butcher like Fleischerei or Metzgerei. In response to newly establishment in October 2013 and enforcement from December 2013 to meat spot sale & processing, a butcher to satisfy hygiene, safety, and equipment standards can now do meat sale business, and produce and sell meat products only by declaration.
Improvement in such a system greatly contributes to the promotion of the 6th industrialization of the pig industry. In other words, as pig producers and meat processors perform primal cut and processing of pork, and directly sell them, primary industry (manufacturing), secondary industry (processing manufacture), and tertiary industry (sales and distribution) need to be carried out together. The 6th industrialization contributes to increase of agricultural income. It also creates job opportunities for elder people and women (Nakano, 2014). In addition, the establishment of the meat spot sale & processing plays a role in expanding consumer’s choice for diversification and upgrading of products to satisfy the desire of colorful consumers. Therefore, it is possible to develop various meat products using low-fat cuts and increase consumption of their products through the 6th industrialization of pig industry.
Conclusions
Recently, increases in income level of consumers, dual-career couple, single households, and the aging population have led to the demand of easy food consumption. Especially, since meat products induce increase of consumption as source of high protein foods, the production of meat products has significantly increased in Korea. The small production and supply systems customized for a consumer become important to supply the processed meat products required by various consumers according to social phenomena apart from existing mass production system. In addition, national agricultural policies must systematically support the agricultural sector internationally as well as the competitiveness of the processing industry through improvement and development of industry technology. Results of this research suggest that, for the 6th industrialization, pig producers and meat processing enterprises need to work together on direct production of raw pork material (primary industry), processing and manufacturing (secondary industry), and distribution or sale (tertiary industry) based on recently established meat spot sale & processing.