Introduction
Beijing You Chicken (BYC) as a precious local breed in Beijing and adjacent areas is famous for high quality and typical appearance with crest, beard, shank feathers and 5 toes (Geng et al., 2018). BYC has become the second livestock and poultry product to obtain the agricultural product geographical indications registration certificate of China after Peking Duck in July 2020. BYC has been well received by local people owing to high nutrition value and unique flavor. The effect of dietary (Qi et al., 2019), photostimulation (Shi et al., 2019) and ranging mode (Geng et al., 2020) were researched on reproduction performance, meat and egg quality of BYC. To protect BYC as a geographical indication, a near-infrared hyperspectral imaging method was used to identify and authenticate BYC breasts among four common chicken breeds (Zhang et al., 2020). BYC has been widely applied to crossbreed with commercial breeds to produce meats or eggs (Ye et al., 2009). Dwarf Beijing You Chicken (DBYC) is a hybrid of purebred BYC and dwarf yellow chicken with smaller body and higher feed efficiency. Both BYC and DBYC eggs had darker yolk color, higher yolk proportion and higher lecithin content than White Leghorn (Tang et al., 2014). It is difficult to distinguish two kinds of eggs in appearance, for the similar eggshell color, shape and size. There was little research available on classification of BYC and DBYC eggs.
Fatty acid, as an important compound of triglycerides, phospholipids and other complex lipids, is one of the energy sources for human beings and an important part of the body biofilm. The total fat content of eggs is about from 30% to 33%, and more than 99% of the fat is in the yolk. Moreover, yolk contains essential unsaturated fatty acids (UFA), including n-3 polyunsaturated fatty acids (PUFA) and n-6 PUFA, which play a very important role in regulating life activities (Kassis et al., 2010). n-3 PUFA could compete enzymes to inhibit and reduce n-6 PUFA (Khatibjoo et al., 2018). Insufficient intake of n-3 PUFA would increase the eicosanoids deriving from n-6 PUFA and lead inflammatory reaction and thrombosis. Excessive amounts of n-6 PUFA and a very high ratio of n-6 polyunsaturated fatty acids to n-3 polyunsaturated fatty acids (n-6/n-3 PUFA) could promote the pathogenesis of many diseases, including cardiovascular disease, cancer, and inflammatory and autoimmune diseases (Wijendran and Hayes, 2004). Intake of proper n-6/n-3 PUFA food is directly related to life and health of human being.
Flavor is a primary parameter determining sensory qualities of eggs and affecting consumers’ choice. Egg breeds, processing methods, storage conditions and diets change composition and content of flavor precursors in eggs, which could affect egg flavor. Zhang et al. (2018) studied flavor compounds of yolks produced by BYC and White Leghorn. He indicated that flavor compounds were related to the characteristics of the breeds. For flavor analysis, solid-phase microextraction (SPME) is known as an effective isolation method. SPME could extract compounds that contribute to food flavor without altering the profile of volatile compounds (Gu et al., 2013). A solid-phase microextraction-gas chromatography-mass spectrometry (SPME-GC-MS) system successfully separated and identified volatile compounds (Zhang et al., 2019). Electronic nose could discriminate slight differences of flavor without subjective factors and judgments, and could be a representative of powerful tools to distinguish flavor profiles. In egg analysis, electronic nose detected fertilization status (Xiang et al., 2019b), TVB-N content (Liu and Tu, 2012), storage time (Yimenu et al., 2017), yolk index (Li et al., 2017) and egg freshness (Dutta et al., 2003). Moreover, SPME-GC-MS and electronic nose were compared to discriminate eggs from different poultry species (Wang et al., 2014).
Classification algorithms, as popular and significant methodologies, meet the demands of different academic disciplines and practical fields. Various types of classification methods, including k-nearest neighbor (KNN), linear discriminant analysis (LDA) (Del Signore, 2001), support vector machine (SVM) and decision tree classification (Pekel, 2020), are performed in pattern recognition and spatial data processing. The overarching objective of this study was to identify the different compounds in BYC and DBYC eggs based on fatty acid profile and flavor characteristics, and discriminate BYC and DBYC eggs based on different classification algorithms. The results of this study would provide theoretical basis and technical support to discriminate BYC eggs as a precious local chicken breed.
Materials and Methods
GC-grade cyclohexanone (C6H10O, ≥99.5% purity, CAS 108-94-1) was purchased from Aladdin Reagents (Shanghai, China). GC-grade n-hexane (C6H14, ≥99.0% purity, CAS 110-54-3) and methyl undecanoate (C12H24O2, ≥98.0% purity, CAS 1731-86-8) were purchased from Macklin Biochemical (Shanghai, China). HPLC-grade methanol (CH3OH, ≥99.9% purity, CAS 67-56-1) was purchased from Mreda Technology (New York, NY, USA). Chemical-grade acetyl chloride (C2H3ClO, ≥99.0% purity, CAS 75-36-5) and analytical-grade potassium carbonate (K2CO3, ≥99.0% purity, CAS 584-08-7) were purchased from Sinopharm Chemical Reagent (Shanghai, China).
A total of 96 eggs per breed of BYC and DBYC provided corn-based feed were gotten from Beijing Animal Husbandry Station (Beijing, China). BYC and DBYC eggs were available in the laboratory with 24 h after being laid and stored at 20±1°C. Fresh eggs without cooking were used for egg quality and fatty acid analyses. Cooked yolks, separated from eggs cooked in the Egg Cooker (Model ZDQ-B07C3, Bear Electric, Foshan, China) for 12 min, were used for SPME-GC-MS and electronic nose analysis. Six fresh eggs without cooking were used for egg quality analysis. Six freeze-dried yolk samples, each consisting of two cooked eggs, were for fatty acid analysis. Three pooled yolk and albumen samples, each consisting of eight cooked eggs, were adopted for SPME-GC-MS measurement. The electronic nose was adopted to test 54 eggs, three measurement replicates of six pooled sample with three birds each.
Egg quality including egg weight, albumen height, yolk color and Haugh unit indexes were detected by multifunctional egg multi tester (Model EA-01, ORKA Food Technology, Ramat Hasharon, Israel).
Fatty acids of yolk samples were analyzed according to the previous method with minor modifications (Ma et al., 2015). Before analysis, about 100 mg freeze-dried yolk samples were weighed and transferred into a 15 mL glass tube. 1 mL n-hexane, 1 mL methyl undecanoate-n-hexane internal standard solution (1 mg/mL) and 4 mL acetyl chloride-methanol solution (10:1) were added to the tube. Then the mixture was methylated in 80°C water for 3 h. After the tube reached room temperature, 5 mL potassium carbonate (7%) was added. The mixture was oscillated and centrifuged at 1,176×g for 5 min and 1.2 mL of upper organic phase was used for analysis. Fatty acids analysis was performed on a gas chromatography-mass spectrometry (GC-MS, Model 7890B-5977B, Agilent Technologies, Santa Clara, CA, USA) equipped with a HP-88 column (100 m×0.25 mm×0.20 μm, Agilent Technologies). 1 μL of sample was injected in a 10:1 split ratio by an autosampler at 250°C injection temperature. The carrier gas was highly pure helium (99.999%) at a constant flow rate of 1 mL/min. The oven temperature was set from 120°C for 1 min, increased at 10°C/min rate up to 175°C for 10 min, then 3°C/min rate up to 210°C for 6 min, finally 2°C/min rate up to 230°C for 6 min. Solvent peak removal time was 3 min. Mass spectrometer scanned mass in the range m/z 50–500. Ion source and interface temperatures were 220°C and 280°C, respectively.
Fatty acids were identified and quantified by comparison with fatty acid standards, which were provided by Sigma Chemical (St. Louis, MO, USA). The relative value of each fatty acid was quantified by computing the peak area ratio of each fatty acid with that of the internal standard. The fatty acid results were demonstrated as milligram fatty acid per gram (mg/g) of yolk. After fatty acid analysis, saturated fatty acids (SFA), UFA, monounsaturated fatty acids (MUFA), PUFA, n-6 PUFA, n-3 PUFA and n-6/n-3 PUFA were calculated.
Volatile compounds of yolk from BYC and DBYC eggs were detected by SPME-GC-MS. The 5 g yolk samples were weighed and placed into a 15 mL headspace vial. For quantitative determination, 1 μL cyclohexanone was dissolved in methanol to get 1 mL internal standard solution, and 30 μL internal standard solution was added to the sample vial. The headspace vial was put in automatic shaker at 60°C for 20 min. Then volatile compounds were extracted at 60°C for 40 min by SPME equipped with a divinylbenzene/carboxen/polymethylsiloxane 50/30 μm fiber (2 cm, DVB/CAR/PDMS, gray, Supelco, PA, USA), and immediately desorbed in a GC-MS injector port at 250°C for 5 min.
The analysis of volatile compounds were performed on a GC-MS (Model 7890B-5977B, Agilent Technologies). The headspace volatiles were separated on a DB-5 column (30 m×0.320 mm×0.25μm, Agilent Technologies) with a helium (99.999%) flow rate of 2.0 mL/min in split-less mode. The running program of oven temperature was 40°C for 3 min, then ramp of 2.5°C/min until 130°C for 3 min, final ramp of 9°C/min to 250°C for 3 min. Mass spectrometric detection was performed in the electron impact mode with an ion source temperature of 230°C, electron energy of 70 eV. The mass scan range was from m/z 35 to 400. Volatile compounds were identified by the mass spectra in the standard NIST 17 library (National Institute of Standards and Technology 14.L, USA) and Wiley library with an acceptance criterion of a score match above 70%. The relative concentration (ng/g) of each volatile compound was quantified by computing the peak area ratio of each compound with that of the internal standard. The volatile compound results were showed as microgram volatile compound per gram of yolk (μg/g).
Yolk flavor was also analyzed by an electronic nose (PEN3, Airsense Company, Schwerin, Germany) equipped with an array of metal oxide semiconductor sensors. The sensory array, applied to distinguish flavor in the sample based on the time response curve, is composed of 10 sensors monitoring the mixture of volatile compounds (Liu and Tu, 2012). 20 g cooked yolk was put in an 15 mL sealed vial, and the parameters of the electronic nose were as follows: sample interval 1 s, flush time 200 s, zero point trim time 10 s, pre-sampling time 5 s, measurement time 120 s, chamber flow 300 mL/min, initial injection flow 300 mL/min (Li et al., 2017; Wang et al., 2014).
Independent t-test was used to determine statistical significant differences at a confidence level of 95% (p<0.05) by SPSS 17.0. The results were presented as mean±SD at least in triplicate. Principal component analysis (PCA)- was performed by Origin 2019b. Classification algorithms, including KNN, LDA, SVM and decision tree, were used to classify BYC and DBYC eggs. Predicted class in models was calculated and compared with true class with 1 for BYC eggs and 0 for DBYC eggs. Classification abilities of different algorithms were shown by classification accuracy. All computations were performed using MATLAB software (2018a, Mathworks, Natick, MA, USA) under the Windows 10 system.
Results
The egg quality of BYC and DBYC eggs is shown in Table 1. The weights of BYC and DBYC eggs were about 53.70 and 52.90 g. The value of yolk color was about 11.40 for BYC eggs and 11.80 for DBYC eggs. No significant difference in egg weight and yolk color was found between BYC and DBYC eggs (p>0.05). The value of albumen height was 5.14 and 5.06 for BYC and DBYC eggs. The value of Haugh unit was 72.60 and 71.60 for BYC and DBYC eggs, respectively. Albumen height and Haugh unit representing egg freshness were not significantly different (p>0.05), indicating that egg freshness between BYC and DBYC eggs were consistent.
Egg quality | BYC | DBYC | p-value |
---|---|---|---|
Weight (g) | 53.70±3.48 | 52.90±2.44 | 0.68 |
Yolk color | 11.40±0.58 | 11.80±0.45 | 0.24 |
Albumen height (mm) | 5.140±0.41 | 5.06±1.04 | 0.88 |
Haugh unit | 72.60±3.14 | 71.60±4.75 | 0.82 |
The fatty acid composition and content in yolk of BYC and DBYC eggs are presented in Table 2. The numbers of fatty acids found in BYC and DBYC yolks were 18 and 20, respectively. The predominant fatty acids, including SFA, MUFA, PUFA, UFA, were palmitic acid (PA, C16:0), linoleic acid (LA, C18:2 n-6), stearic acid (SA, C18:0), arachidonic acid (ARA, C20:4 n-6), hexadecenoic acid (C16:1 n-7), oleic acid (OA, C18:1 n-9) and docosahexaenoic acid (DHA, C22:6 n-3). Statistical analysis demonstrated that content of MUFA, PUFA, and UFA was also significantly different between BYC and DBYC yolks (p<0.05). The content of n-6 PUFA for BYC and DBYC yolks was 43.28 and 49.12 mg/g. The n-3 PUFA content of BYC and DBYC yolks was 6.77 and 8.34 mg/g. The n-6/n-3 PUFA showed no differences with 6.58 and 6.33 for BYC and DBYC yolks.
BYC, Beijing You Chicken; DBYC, Dwarf Beijing You Chicken; SFA, saturated fatty acids; MUFA, monounsaturated fatty acids; LA, linoleic acid; GLA, γ-linolenic acid; ARA, arachidonic acid; n-6 PUFA, n-6 polyunsaturated fatty acids; ALA, α-linolenic acid; ND, not detected; EPA, eicosapentaenoic acid; DPA, docosapentaenoic acid; DHA, docosahexaenoic acid; n-3 PUFA, n-3 polyunsaturated fatty acids. PUFA, polyunsaturated fatty acids; UFA, unsaturated fatty acids; n-6/n-3 PUFA, the ratio of n-6 polyunsaturated fatty acids to n-3 polyunsaturated fatty acids.
For BYC and DBYC yolks, a total of 47 volatile compounds were identified. As shown in Fig. 1A and 1B, the volatile compounds were divided into 11 categories, including 10 ketones, 9 N-containing compounds, 4 acids, 5 esters, 4 aldehydes, 4 aromatics, 3 alcohol, 1 alkanes, 2 alkene, 1 S-containing and 4 others. The relative concentration of volatile compounds in BYC and DBYC yolks are presented in Table 3. The volatile component content of 11 categories in BYC and DBYC yolk is summarized in Fig. 1C. Ketones combined with N-containing ketones were the largest category, presenting approximately 50% and 60% of the total volatile compounds for BYC and DBYC yolks. Ketones, attributed to free fatty acid oxidation, amino acid degradation and Maillard reaction, were the main source of yolk bad smell. Among 10 ketones, 7 had significant differences between BYC and DBYC yolks (p<0.05). The 2-hydroxy-2-cyclopenten-1-one had the highest content in BYC yolks, while the content of cyclohexanone were the highest one in DBYC yolks. Alkenes, the third largest category, had significant differences in BYC and DBYC yolks (p<0.05). 3-methyl-1-pentene was the main representative alkenes in BYC and 4-ethyl-3-heptene was in DBYC. The alkenes, alkanes and aromatics might be metabolites of triglyceride degradation and fatty acid oxidation, such as LA oxidation. Different content of fatty acids in BYC and DBYC yolks would explain the significant differences of 3-methyl-1-pentene, toluene and 4-ethyl-3-heptene in alkenes group. Among 5 esters, 4 had significant differences between BYC and DBYC yolks (p<0.05). Benzoic acid, 2-phenylethyl ester had higher content in BYC yolks. While for DBYC yolks, the third largest group was aromatic group. For acid category, 2 of 4 acids were significantly different between BYC and DBYC yolks (p<0.05). Aldehydes with low odor thresholds and low molecular weights could play an important role in egg flavor (Gouda et al., 2019). Hexanal and 2-ethyl-hexanal had significant differences between BYC and DBYC yolks (p<0.05). The saturated aldehydes might come from the thermal derivative, oxidation degradation of fatty acids in yolks and the Strecker reaction of the amino acid. On the whole, 35 of 47 volatile compounds were significantly different between BYC and DBYC yolks (p<0.05).
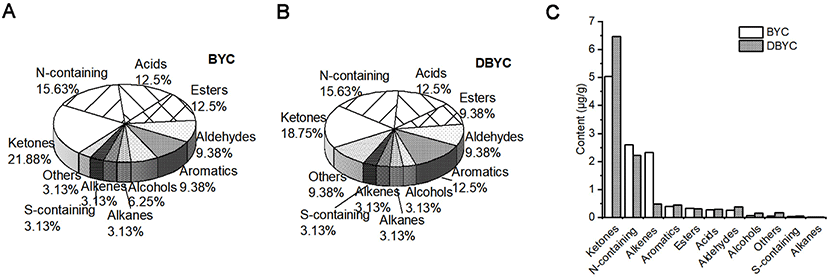
For BYC and DBYC eggs, electronic nose radar image with 10 sensors on behalf of the average signal variation for every sensor is shown in Fig. 2A. Sensors W1W, W2W, W1S, W2S, and W5S displayed higher values than the rest of the sensors, with W1W exhibiting the highest values. Contribution rate of the first principal component (PC1) and second principal component (PC2) in Fig. 2B were 65.78% and 30.07%, respectively. The total accumulative contribution rate of PC1 and PC2 reached 95.85%. As displayed in Fig. 2C, BYC and DBYC eggs would be well classified by PCA. The PCA loading plot, which revealed compounds in charge of egg flavor, is also illustrated in Fig. 2C. Sensors W5S and W1W with values more than 0.35 were on the right side of PC1. Sensors W5C and W1C with values less than –0.35 were on the left side of PC1. Sensor W3S, W1S, W6S, and W2S had higher loading value on PC2.
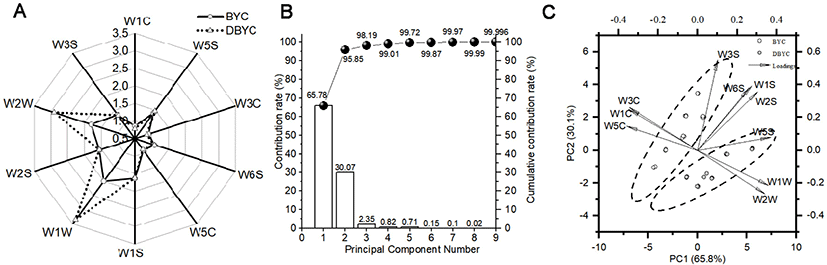
The classification accuracy of KNN, LDA, SVM and decision tree was presented in Table 4. For fatty acid data, both KNN and SVM got better classification accuracy with 91.7% than LDA with 83.3% and decision tree with 50%. For SPME-GC-MS data, LDA failed to class BYC and DBYC eggs, SVM and decision tree got 16.7% and 33.3% classification accuracy. The highest accuracy was only 50% based on KNN. For electronic nose data, KNN, LDA, SVM and decision tree classification algorithms all obtained 100% accuracy.
Classification algorithm | Fatty acid | Volatile compound | |
---|---|---|---|
SPME-GC-MS | Electronic nose | ||
KNN | 91.7 | 50.0 | 100 |
LDA | 83.3 | - | 100 |
SVM | 91.7 | 16.7 | 100 |
Decision tree | 50.0 | 33.3 | 100 |
Discussion
The weights of BYC and DBYC eggs were about 53.70 and 52.90 g, lower than the other breeds, such as Issa Brown pullet eggs ranging from 65.77 to 62.62 g (Ayerza and Coates, 1999) and Hy-line Brown laying hen eggs from 61.00 to 61.30 g (Orczewska-Dudek et al., 2020). In previous studies, weights of BYC eggs were about 40.30 g (Zheng et al., 2019), from 47.64 to 56.93 g (Geng et al., 2018) and from 42.48 to 46.34 g (Geng et al., 2020). BYC egg weights might varied with dietary, ranging mode, lighting pattern or stocking density. Yolk color was about 11.40 for BYC eggs and 11.80 for DBYC eggs. Yolk colors measured by another egg multi tester were 7.18 in control group (Zheng et al., 2019), from 5.6 to 7.67 in different lighting modes (Geng et al., 2018) and from 7.49 to 8.54 with different indoor stocking densities (Geng et al., 2020). Moreover, the egg multi testers from different instrument companies would also influence the yolk color results. Egg quality did not reveal any significant difference in albumen height and Haugh unit in agreement with previous researches (Wen et al., 2019). The consistent egg freshness made sure that following researches about fatty acid and flavor compounds in BYC and DBYC eggs were mainly influenced by breeds.
The fatty acid composition of yolk was consistent with previous studies (Lawlor et al., 2010). DBYC yolks had higher content of arachidic acid, OA, eicosatrienoic acid (C20:3 n-6) and docosapentaenoic acid (DPA, C22:5 n-3) than BYC yolks (p<0.05). While hexadecenoic acid (C16:1 n-7) content in DBYC yolks was lower than that of BYC yolks (p<0.05). The content of MUFA, PUFA, and UFA was also significantly different between BYC and DBYC yolks (p<0.05). In many studies, fatty acid results were shown as percentage content of total fatty acids (%). The PUFA content was higher than that of line 477 chicken yolks with 18.04% in control diet (Baeza et al., 2015). The content of n-6 PUFA for BYC and DBYC yolks was lower than those of White Bovan yolks with 68.50 mg/g. The n-3 PUFA content of BYC and DBYC yolks was higher than that of White Bovan yolks with 2.40 mg/g in control group, which ranged from 6.00 to 15.30 mg/g by diets with Hemp or Hempseed oil (Goldberg et al., 2012). It is known that proper n-6/n-3 PUFA could help to mitigate effects of various diseases, and also can promote ongoing health and vitality of human consumers (Wang et al., 2019). The n-6/n-3 PUFA was much lower than those of line 477 hen yolks with 15.20 in control group (Baeza et al., 2015) and White Bovan yolks with 28.54 (Goldberg et al., 2012). The n-6/n-3 PUFA was dropped to 6.20, 5.72, 4.20, and 4.90 in extruded linseed with a high-crude fiber content (ELHF), extruded linseed with a low-crude fiber content (ELLF), microalgae (MA) and an association of 75% ELLF and 25% MA groups (Baeza et al., 2015). In conclusion, the low n-6/n-3 PUFA in BYC and DBYC yolks supplied potential benefits to human health.
A total of 47 volatile compounds were identified in BYC and DBYC yolks, including 10 ketones, 9 N-containing compounds, 4 acids, 5 esters, 4 aldehydes, 4 aromatics, 3 alcohol, 1 alkanes, 2 alkene, 1 S-containing and 4 others. Ianni et al. (2020) found that analysis of the volatile profile could not identify any compound in yolks from ISA Warren laying hens with or without a dietary supplementation of extruded linseed. Xiang et al. (2019b) reported that 14 volatile compounds, containing nonanal, decanal, 6-methyl-5-hepten-2-one and 6,10-dimethyl-5,9-undecadien-2-one, were found in unhatched shell White Leghorn eggs based on SPME-GC-MS. Later, they discovered that a total of 17 or 18 volatile compounds, including nonanal, decanal and 6-methly-5-hepten-2-one, were identified in White Leghorn, Hy-line Brown and Jing Fen hatching eggs. Heptanal, 6-methly-5-hepten-2-one and octanal had positive contribution in classifying of White Leghorn, Hy-line Brown and Jing Fen hatching eggs (Xiang et al., 2019a). 29 volatile compounds with 2-acetyl-1-pyrroline, 2,3-butanedione, hexanal, heptanal and 1-hexanoland methional as major contributors were divided into 7 categories consisting of alcohol, ketones, esters, aldehydes, hydrocarbon, phenolic and furan derivatives in whey protein isolate with egg yolk addition (Paraskevopoulou et al., 2014). Gouda et al. (2019) reported that 111 different volatile compounds were found in yolks with trans-cinnamaldehyde, thymol, menthol and vanillin additions by using SPME-GC-MS. While in the control group without bio-active volatile terpenes and natural compounds, only 48 volatile compounds were reported and alcohols including 3-phenyl-2-propyn-1-ol, phytol, levomenthol and pentadecanoyl accounted for almost 75%. Inconsistencies between volatile compounds identified by SPME-GC-MS would be influenced by egg breeds, cooking time and test parameters.
Electronic nose detection with good reproducibility and repeatability, is a simple, quick, nondestructive and specific technique. Electronic nose is adopted for measuring the volatile fingerprint based on the time responses of sensor arrays, while could not identify flavor compounds as precisely as SPME-GC-MS (Qin et al., 2020). Sensors W1W, W2W, W1S, W2S, and W5S were more important on BYC and DBYC egg detection. It was clear that sensors W1W and W2W, delegating sulfur, aromatic and sulfur organic compounds, had obvious differences. PCA was used to reduce the dimension of data, transform the original variable data, eliminate the overlapping parts in the coexistence of many information. PCA is an unsupervised technique for classifying BYC and DBYC eggs based on the inherent similarity or dissimilarity of their chemical information without prior knowledge of egg classes. PC1 and PC2 could be used as representatives for subsequent analysis. BYC and DBYC eggs would be well classified by PCA. For PC1, sensors W5S and W1W indicating nitrogen oxides and sulfur organic had the main positive contribution, sensors W5C and W1C indicating alkane and aromatic had the main positive contribution. For PC2, sensor W3S, W1S, W6S, and W2S, had the positive influence on PC2. On the whole, sensors W5S, W5C, W1W, and W3S had higher influence in PCA for BYC and DBYC egg classification. While sensors W1C, W5S, W3C, W5C, W1S, and W2S had greater effect on discrimination of Brown Hy-line eggs based on storage periods (Liu and Tu, 2012). This could be interpreted that main sensors would change with egg breeds and indexes.
In classification algorithms, the total ion chromatogram data of fatty acids and SPME-GC-MS volatile compounds was analyzed without considering specific composition or content corresponding to the curves. The time response data of electronic nose was directly used in classification analysis, not regarding sensitive characteristics of 10 sensors. This would save calculation and analysis time, ensure the accuracy of classification results avoiding impact of specific substance misjudgment. For fatty acid data, both KNN and SVM got better classification accuracy. For SPME-GC-MS data, all algorithms got poor classification accuracy. Based on electronic nose data, LDA could classify eggs for egg storage time and SVM was applied to build prediction models of yolk index with square correlation coefficient of 0.9641 in training set and 0.8339 in testing set (Li et al., 2017). PCA was reported to get better results than LDA to distinct pure and adulterated honey samples based on electronic nose (Subari et al., 2012). Different results for the same index data could explain by algorithm theory. KNN classification algorithm checks the distance between a test sample and a training sample. According to the distance between them and the selected point, LDA classes data points which are projected onto a straight line to reduce the dimension of the data set. The principle of SVM is to draw a line between different data point clusters and group them into some classes. Decision tree is computed by decomposing the data set into smaller and smaller subsets according to different criteria. For electronic nose data, KNN, LDA, SVM and decision tree classification algorithms all obtained best results. This indicated that classification results also varied according to detection index.
Conclusion
BYC and DBYC eggs were analyzed to find differences in fatty acids and volatile compounds, and classified combined with classification algorithms including KNN, LDA, SVM and decision tree. For fatty acid profile, arachidic acid, OA, eicosatrienoic acid and DPA, hexadecenoic acid, MUFA, PUFA, and UFA were significantly different between BYC and DBYC yolks (p<0.05). For flavor characteristics, a total of 47 volatile compounds were identified in BYC and DBYC yolks by SPME-GC-MS. In which, 35 volatile compounds, comprising 2-hydroxy-2-cyclopenten-1-one and cyclohexanone, had significant differences between BYC and DBYC yolks (p<0.05). Based on classification algorithms, the fatty acid data got better classification accuracy with 91.7% by KNN and SVM, while SPME-GC-MS displayed poor classification results. Furthermore, electronic nose data could be classified well according to egg breeds by PCA and got 100% classification accuracy by KNN, LDA, SVM and decision tree. These results showed BYC and DBYC eggs could be classified based on electronic nose with appropriate classification algorithms. This study could be used to distinguish different varieties of eggs, protect local unique varieties and prevent counterfeiting. These results also enlighten to identify characteristics and classify multiple complex samples in the future.